AI Technique Designed to Advance Understanding of Neural Circuits
Scientists from Ecole Polytechnique Fédérale de Lausanne (EPFL) and Harvard have developed an AI method to track neurons inside moving and contorted animals. The study, titled "Automated neuron tracking inside moving and deforming animals using deep learning and targeted augmentation," led by Sahand Jamal Rahi, PhD, at EPFL’s School of Basic Sciences, was published in Nature Methods. The researchers addressed the challenge of reading neuronal activity from 3D functional imaging in behaving animals where the brain moves and deforms. Their innovative approach involves training a convolutional neural network with ground-truth annotations of images representing various brain postures.
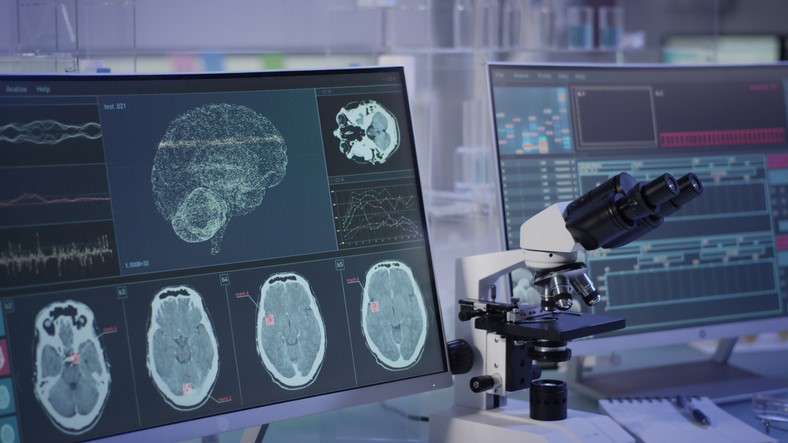
Figure 1. AI Technique Designed to Advance Understanding of Neural Circuits
Figure 1 shows AI Technique Designed to Advance Understanding of Neural Circuits The researchers acknowledge the labor-intensive nature of annotating 3D images and propose a solution called targeted augmentation. This method automatically generates synthetic annotations from a limited number of manual annotations. The developed technique, named 'Targettrack,' learns the internal deformations of the brain, allowing it to synthesize annotations for new postures by deforming the ground-truth annotations. This approach significantly reduces the manual annotation and proofreading workload.
The researchers have implemented a graphical user interface for the end-to-end application of Targettrack. They demonstrate its effectiveness on recordings where neurons are labeled as key points or 3D volumes. By analyzing freely moving animals exposed to odor pulses, the team uncovers intricate patterns in interneuron dynamics, including the ability to switch neuronal entrainment on and off. The breakthrough achieved with Targettrack has the potential to expedite research in brain imaging and contribute to a deeper understanding of neural circuits and behaviors, according to Rahi.
Neural Network Using Convolutions
The newly developed technique relies on a convolutional neural network (CNN), a form of artificial intelligence trained to recognize patterns in images. The CNN uses a process called "convolution," which involves analyzing small portions of an image, such as edges, colors, or shapes, and combining this information to identify objects or patterns.
The challenge in identifying and tracking neurons in a moving animal's brain arises because the animal's appearance changes over time due to various body contortions in a movie. Manual labeling of numerous images is typically required for this process. However, due to the diverse postures of the animal, generating a sufficient number of annotations manually for CNN training can be a formidable task.
To overcome this challenge, the researchers developed an advanced CNN incorporating targeted augmentation. This method automatically generates annotations for reference from a limited set of manual annotations. The CNN learns the internal contortions of the brain and uses this knowledge to create annotations for new postures. This innovation significantly reduces the necessity for manual annotation and verification processes.
The newly developed method demonstrates its versatility by successfully identifying neurons represented as individual points or 3D volumes in images. The researchers conducted tests using the roundworm, Caenorhabditis elegans, a widely studied model organism in neuroscience due to its 302 neurons.
Employing the enhanced CNN, the scientists focused on measuring the activity of some of the worm's interneurons. The results revealed complex behaviors, such as changes in response patterns when exposed to various stimuli, including periodic bursts of odors. To facilitate broader adoption, the research team has made their CNN accessible through a user-friendly graphical interface. This interface incorporates targeted augmentation, streamlining the entire process into a comprehensive pipeline—from manual annotation to final proofreading, as highlighted by Rahi.
Source:genengnews
Cite this article:
Janani R (2023), AI Technique Designed to Advance Understanding of Neural Circuits, AnaTechMaz, pp.390