AI Model Weighs Epigenetic Factors to Predict Cancer Outcomes
Researchers from the UCLA Health Jonsson Comprehensive Cancer Center have developed an artificial intelligence (AI) model that utilizes tumor-associated epigenetic factors to successfully predict patient outcomes across various types of cancer. The study involved analyzing tumor gene expression patterns of epifactors—epigenetic factors influencing gene activation or repression. By categorizing tumors into distinct groups based on these patterns, the AI model outperformed traditional measures like cancer grade and stage in predicting patient outcomes across different cancer types. The findings pave the way for targeted anticancer therapies focused on regulating epigenetic factors, including histone acetyltransferases and SWI/SNF chromatin remodelers. The researchers believe their work provides a roadmap for generating similar AI models using publicly available lists of prognostic epigenetic factors, offering potential insights for predicting specific cancer treatment targets.
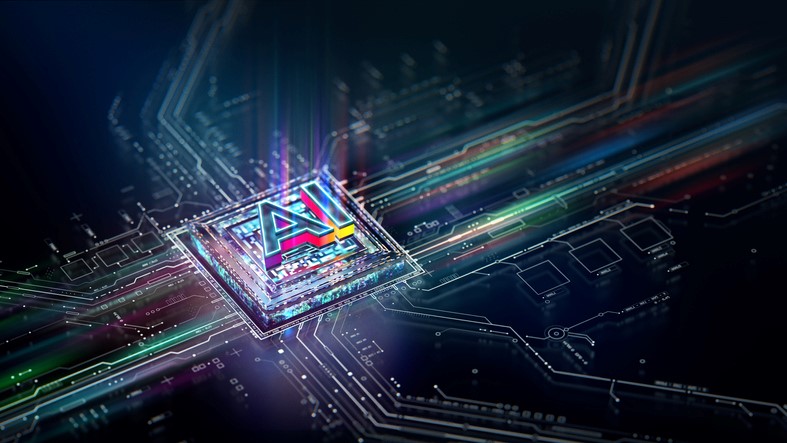
Figure 1. AI Model Weighs Epigenetic Factors to Predict Cancer Outcomes
Figure 1 Shows AI Model Weighs Epigenetic Factors to Predict Cancer Outcomes A team of researchers, co-led by Dr. Hilary Coller, professor of molecular, cell, and developmental biology and a member of the UCLA Health Jonsson Comprehensive Cancer Center and the Eli and Edythe Broad Center of Regenerative Medicine and Stem Cell Research at UCLA, has published a paper titled "Pan-cancer landscape of epigenetic factor expression predicts tumor outcome." The study delves into the realm of epigenetics, focusing on protein factors and processes that govern the establishment and maintenance of different genomic states, including gene activity levels. The researchers utilized advanced next-generation sequencing technologies to create an epigenetic map of various cancer types, paving the way for the discovery of pan-cancer targetable epifactors.
Epigenetic processes involve changes such as DNA methylation, histone protein modifications, chromatin accessibility, and higher-order chromatin architecture. These changes are mediated by chromatin-associated protein factors, also known as epigenetic factors or epifactors, which play a crucial role in cancer and its progression. Dr. Coller emphasized that understanding differences in the chromatin state among tumors can offer insights into variations in patient responses to treatments and outcomes. The researchers noted that epifactors themselves can undergo genetic alterations in tumors, leading to widespread epigenetic dysregulation. This study contributes to advancing the understanding of the role of epigenetics in cancer and highlights the potential for targeting epifactors across different cancer types.
Previous studies have established that mutations in genes encoding epigenetic factors can influence cancer susceptibility, but the impact of these factors' levels on cancer progression remains poorly understood. The researchers, led by Dr. Hilary Coller, addressed this gap by leveraging The Cancer Genome Atlas (TCGA) to analyze the expression patterns of 720 epigenetic factors across 24 different cancer types. Using the non-negative matrix factorization algorithm, they classified tumors into distinct clusters based on the most variably expressed epifactor genes.
The study revealed that out of the 24 adult cancer types studied, clusters formed by epigenetic patterns were associated with significant differences in patient outcomes for 10 cancers. These differences included progression-free survival, disease-specific survival, and overall survival. Notably, epifactors influencing poor outcomes were correlated with higher cancer stage, larger tumor size, or more severe spread indicators. The researchers also found that the prognostic efficacy of an epigenetic factor was influenced by the tissue-of-origin of the cancer type. This information may be valuable in assessing the cancer-specific relevance of therapeutically targeting these factors, offering insights into potential avenues for personalized cancer treatments.
The research team utilized the gene expression levels of epigenetic factors to train and test an artificial intelligence (AI) model, employing machine learning to predict patient outcomes across five cancer types. This pan-cancer AI model successfully categorized patients into groups with significantly different chances of better or poorer outcomes, demonstrating its predictive power. The model was specifically designed for cancers that exhibited significant differences in survival measurements.
Furthermore, the researchers identified genes crucial for the AI model, and these genes significantly overlapped with the cluster-defining signature genes. The pan-cancer AI model was trained and tested on adult patients from The Cancer Genome Atlas (TCGA) cohort, emphasizing the potential for broad applicability across different datasets. The authors suggested the exploration of similar epigenetic factor-based models for pediatric cancers to understand the factors influencing decision-making in comparison to models built on adult cancers.
The study also highlighted the potential therapeutic implications of their findings. Numerous epifactors, including enzymes involved in DNA methylation, histone methylation, and histone acetylation, were identified as potential targets for anti-cancer therapy. Notably, histone acetyltransferases were enriched among prognostic genes associated with improved patient outcomes, supporting the potential benefits of histone deacetylase inhibitors in cancer treatment. Additionally, the SWI/SNF family of chromatin remodelers emerged as possible targets for epigenetics-based cancer therapy. The authors concluded that their pan-cancer study contributes to understanding clinical differences between cancer types and provides a foundation for rational drug design targeting epigenetic regulators.
Source:genengnews
Cite this article:
Janani R (2023), AI Model Weighs Epigenetic Factors to Predict Cancer Outcomes, AnatechMaz, pp.387