The Power of Deep Learning in X-ray Diffraction Analysis for Advanced Materials Characterization
In a groundbreaking study published in npj Computational Materials, scientists from the University of Rochester reveal how deep learning can revolutionize the analysis of X-ray diffraction experiments, a method already considered the gold standard for characterizing new materials. Led by project lead Niaz Abdolrahim, an associate professor in the Department of Mechanical Engineering, the interdisciplinary team developed innovative models to harness the vast amounts of data produced during X-ray diffraction experiments.
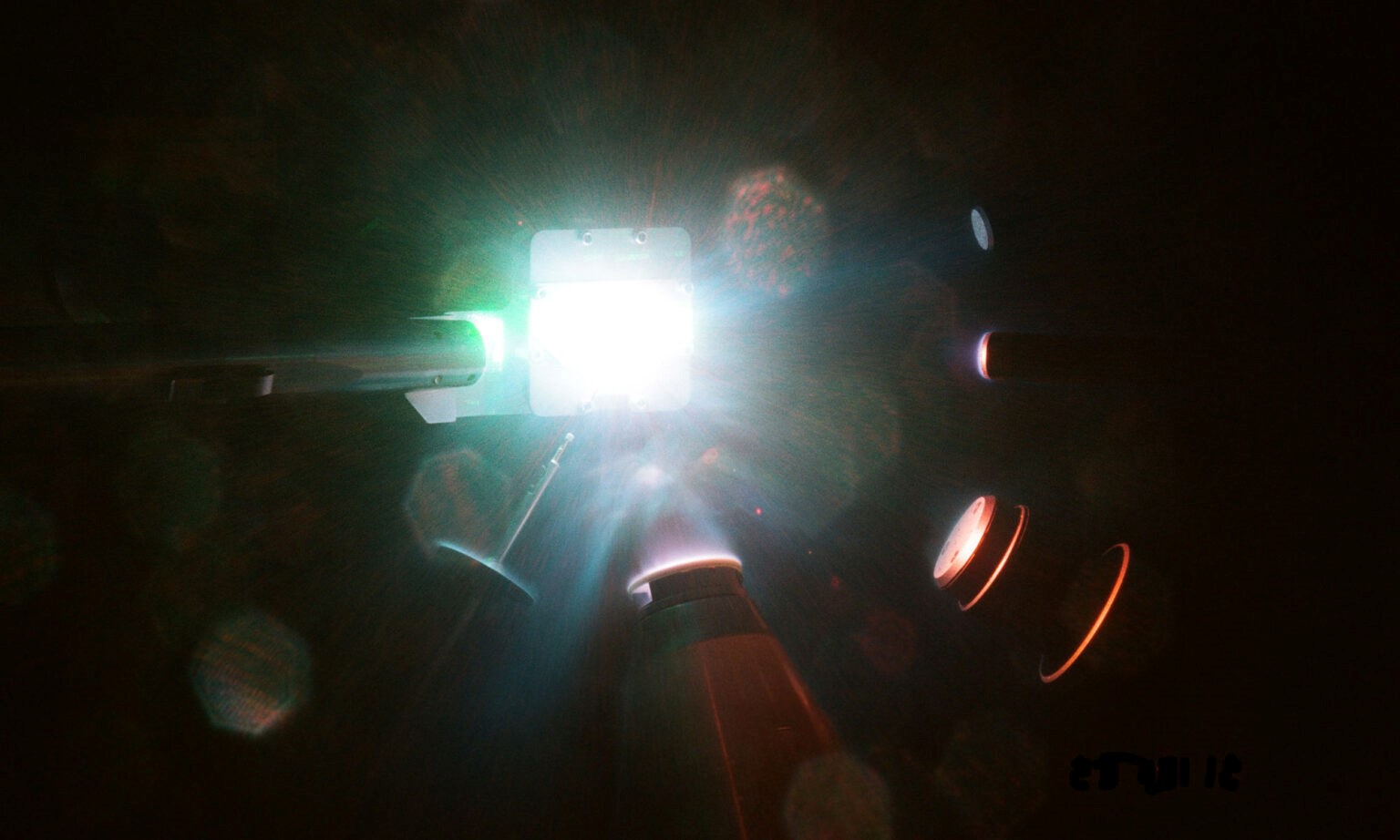
Figure 1. Material on a powder X-ray diffraction image plate. (Credit: Danae Polsin and Gregory Ameele)
Figure 1 shows material on a powder X-ray diffraction image plate is analyzed at the Omega Laser Facility at the University of Rochester’s Laboratory for Laser Energetics. Scientists are developing deep-learning models to analyze the massive amounts of data produced by these experiments.
The Challenge
X-ray diffraction experiments involve shining bright lasers on a sample, generating diffracted images containing crucial information about a material's structure and properties. Abdolrahim emphasizes that traditional methods of analyzing these images are often contentious, time-consuming, and ineffective. With terabytes of data generated daily worldwide, developing advanced models becomes essential for accelerating materials innovation, understanding materials at extreme conditions, and creating materials for diverse technological applications.
The Promise for High-Energy-Density Experiments
The study, spearheaded by Jerardo Salgado '23 MS in materials science, holds particular promise for high-energy-density experiments, such as those conducted at the Laboratory for Laser Energetics (LLE). By precisely examining the moments when materials under extreme conditions change phases, scientists can unlock insights into creating new materials and gain knowledge about the formation of stars and planets.
Real-World Data Integration
Abdolrahim highlights that the project improves upon previous attempts to develop machine learning models for X-ray diffraction analysis. Unlike models primarily trained and evaluated with synthetic data, the team, including Associate Professor Chenliang Xu from the Department of Computer Science, incorporated real-world data from experiments with inorganic materials to train their deep-learning models.
The Path Forward
While celebrating their success, Abdolrahim stresses the importance of making more X-ray diffraction analysis experimental data publicly available. The team is actively working on creating platforms for sharing data, inviting collaboration to further refine and evaluate their system, ultimately enhancing its effectiveness.
The integration of deep learning into X-ray diffraction analysis marks a significant leap forward in materials science. The University of Rochester's interdisciplinary team's commitment to using real-world data sets and fostering collaboration demonstrates a dedication to advancing this cutting-edge technology, with potential implications for materials innovation, extreme condition research, and the exploration of celestial formations.
Source: University of Rochester
Cite this article:
Hana M (2024), The Power of Deep Learning in X-ray Diffraction Analysis for Advanced Materials Characterization, AnaTechMaz, pp. 376