Advancements in Causal Machine Learning for Medical Applications
An international research team, led by Ludwig-Maximilians-Universität München (LMU) and including University of Cambridge researchers, is delving into the potential of a relatively new facet of AI for medical diagnostics and therapy. Their study, published in Nature Medicine, highlights the promise of causal machine learning (ML) in estimating treatment outcomes more effectively than conventional ML methods. This advancement could lead to safer, more efficient, and personalized medical treatments.
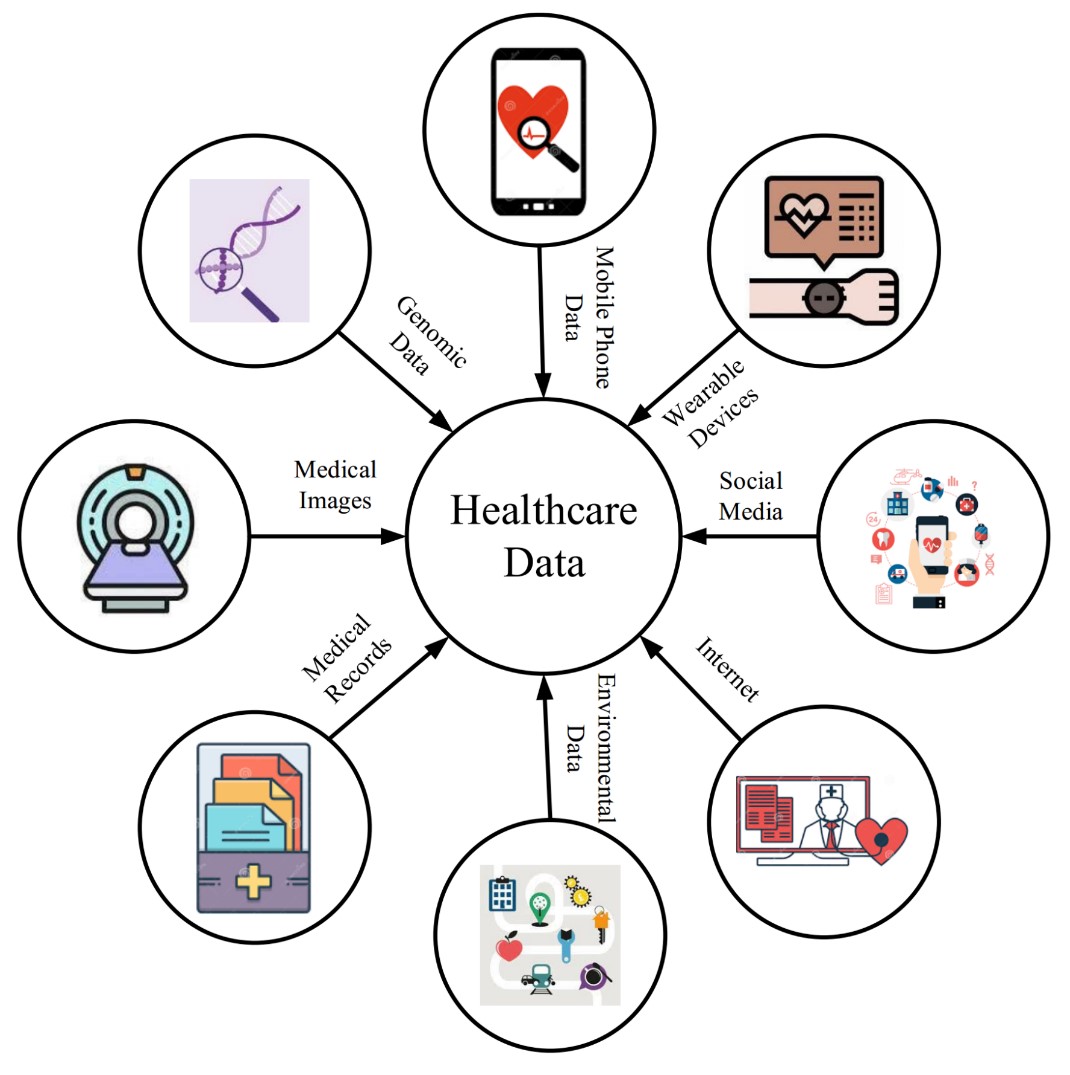
Figure 1. ML in medical applications.
Figure 1 shows ML in medical applications. While traditional ML excels at recognizing patterns and correlations in data, it typically falls short in understanding cause-and-effect relationships—crucial for informed medical decision-making. Causal ML, however, addresses this gap by enabling clinicians to personalize treatment strategies based on a deeper understanding of causal relationships.
For instance, in assessing treatment options for individuals at risk of diabetes, causal ML goes beyond predicting disease likelihood by evaluating the impact of specific interventions, such as anti-diabetes medication like metformin. By answering "what if?" questions, these models can estimate the effects of hypothetical treatments, even in scenarios where conventional randomized studies are not feasible [1].
However, implementing causal ML in medicine requires tailored modeling and close collaboration between AI experts and medical professionals. While these methods have been tested in other fields like marketing, adapting them for clinical use involves unique challenges and opportunities.
Professor Mihaela van der Schaar, the senior author of the study, underscores the significance of this progress after years of dedicated research. Moving forward, she emphasizes the importance of validating these tools across various clinical contexts, from transplantation to cancer and cardiovascular disease, to ensure their reliability and efficacy in real-world settings.
Source: University of Cambridge
References:
- https://www.nature.com/articles/s41591-024-02902-1
Cite this article:
Hana M (2024), Advancements in Causal Machine Learning for Medical Applications, AnaTechMaz, pp. 381