Accelerating Parkinson’s Drug Discovery with AI
Researchers at the University of Cambridge employed an AI-driven approach to pinpoint compounds that hinder the aggregation of alpha-synuclein, a protein associated with Parkinson’s disease. By leveraging machine learning techniques, they swiftly screened a vast chemical library, identifying five potent compounds for further exploration. This advancement is crucial given the rising prevalence of Parkinson’s, which currently lacks effective disease-modifying treatments. Traditional screening methods are both time-consuming and costly, often yielding minimal success.
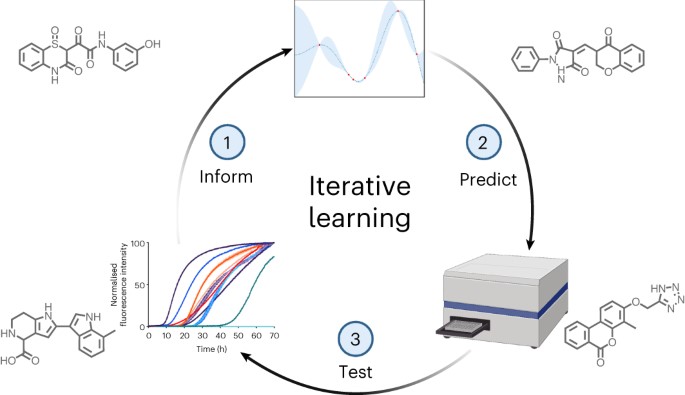
Figure 1. Iterative Learning. (Credit: Robert I Horne et al)
Figure 1 shows iterative learning. Parkinson’s is a rapidly growing neurological disorder, with projections indicating a tripling of cases by 2040. In the UK alone, the likelihood of a Parkinson’s diagnosis is one in 37 [1]. Beyond its motor symptoms, Parkinson’s adversely affects various bodily systems, contributing to a diminished quality of life.
The pathology of Parkinson’s involves proteins, like alpha-synuclein, malfunctioning and forming harmful clusters known as Lewy bodies, leading to nerve cell death. Targeting these aberrant proteins presents a promising avenue for treatment [1]. However, the conventional process of identifying potential therapies is arduous, taking months or even years to yield viable candidates.
The Cambridge team’s breakthrough lies in their innovative machine learning approach, which streamlines the screening of chemical libraries, significantly reducing time and costs [2]. By employing computational screening, they expedited the identification of molecules capable of inhibiting alpha-synuclein aggregation. This method involved iterative feedback between computational predictions and experimental validation, leading to the discovery of highly potent compounds [2].
Through their approach, the researchers honed in on specific regions of small molecules responsible for binding to amyloid aggregates, significantly enhancing their efficacy. These compounds target critical sites on aggregate surfaces, disrupting their proliferation. Notably, these newly developed compounds exhibit hundreds of times greater potency and are more cost-effective than previously reported options.
The integration of machine learning into drug discovery represents a paradigm shift, accelerating the identification of promising candidates. For the Cambridge team, this means embarking on multiple drug discovery initiatives concurrently, fueled by substantial reductions in time and costs. This advancement underscores the transformative potential of AI in driving therapeutic innovation.
The research was conducted within the Chemistry of Health Laboratory at Cambridge, supported by the UK Research Partnership Investment Fund (UKRPIF), emphasizing the importance of translating academic research into clinical applications.
Source: University of Cambridge
References:
- https://www.nature.com/articles/s41589-024-01580-x#citeas
- https://www.m3india.in/contents/clinical_news/ai-speeds-up-drug-design-for-parkinson-s-by-ten-fold
Cite this article:
Hana M (2024), Accelerating Parkinson’s Drug Discovery with AI, AnaTechMaz, pp. 379