Edge AI
Edge AI, also known as AI at the edge, refers to the deployment of AI algorithms and models on edge devices, such as smartphones, sensors, cameras, and IoT devices, rather than in the cloud or a central server. The goal of edge AI is to bring AI processing closer to the source of the data, reducing latency and bandwidth requirements, enabling faster decision-making, and improving the privacy and security of data.
Edge AI typically involves deploying lightweight, low-power AI models that can run efficiently on resource-constrained edge devices. These models are optimized for the specific hardware and software architecture of the edge device, allowing them to run faster and with less power consumption than traditional AI models.
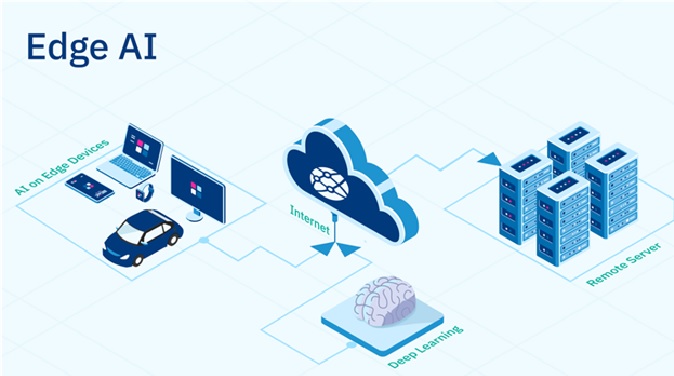
Figure 1. Edge AI
Figure 1 shows Edge AI. Some common applications of edge AI include:
- Object detection and recognition: Edge AI algorithms can be used to detect and recognize objects in real-time, enabling devices such as cameras and drones to respond quickly to changing conditions.
- Predictive maintenance: Edge AI algorithms can analyze sensor data in real-time to predict when equipment is likely to fail, enabling organizations to perform maintenance before a failure occurs.
- Autonomous vehicles: Edge AI algorithms can be used to process sensor data in real-time, enabling autonomous vehicles to make decisions quickly and accurately.
- Health monitoring: Edge AI algorithms can be used to analyze data from wearables and other health monitoring devices in real-time, enabling early detection of health problems and personalized treatment.
- Natural language processing: Edge AI algorithms can be used to process natural language queries in real-time, enabling devices such as smart speakers and chatbots to respond quickly to user requests.
There are several benefits of Edge AI, including:
- Reduced Latency: Edge AI algorithms can process data locally on the edge devices, reducing the time it takes to send data to a central server for processing and back. This reduced latency enables faster decision-making, making edge AI ideal for real-time applications that require immediate responses, such as autonomous vehicles or industrial automation.
- Improved Data Privacy: By processing data locally on edge devices, Edge AI reduces the need to send data to a central server for processing, which can help to maintain data privacy and security. This is particularly important in applications that involve sensitive data, such as healthcare or finance.
- Increased Efficiency: Edge AI algorithms are designed to run on resource-constrained devices, making them more efficient than traditional AI models that require large amounts of computing power and storage. This increased efficiency reduces the cost of deploying AI solutions and makes them more accessible to a wider range of applications and industries.
- Reduced Bandwidth Requirements: Edge AI algorithms can process data locally, reducing the amount of data that needs to be transmitted to a central server for processing. This reduces the bandwidth requirements and can help to reduce the cost of data transmission.
- Increased Reliability: Edge AI can improve the reliability of applications by reducing dependence on a central server for processing. If the connection to the central server is lost, Edge AI can continue to function, ensuring that critical applications continue to operate.
- Improved Scalability: Edge AI can be deployed on a large number of devices, making it ideal for applications that require scalability, such as smart cities or industrial automation. This allows organizations to deploy AI solutions more quickly and at a lower cost than traditional AI models.
Overall, edge AI is an emerging field with significant potential for innovation and disruption in various industries. As edge devices become more powerful and capable, we can expect to see more sophisticated and powerful AI applications deployed at the edge.
References:
- https://www.cdotrends.com/story/16179/ai-edge-enabling-digital-transformation
Cite this article:
Hana M (2023), Edge AI, AnaTechMaz, pp.218