Multimodal Learning
Multimodal learning involves combining different types of data, such as images, text, and audio, to improve the accuracy of computer vision systems. This has applications in fields such as natural language processing, where multimodal models can be used to generate more accurate and context-aware responses.
Multimodal learning in machine learning is a type of learning where the model is trained to understand and work with multiple forms of input data, such as text, images, and audio. These different types of data correspond to different modalities of the world – ways in which it’s experienced. The world can be seen, heard, or described in words. For a ML model to be able to perceive the world in all of its complexity and understanding different modalities is a useful skill. For example, let’s take image captioning that is used for tagging video content on popular streaming services. The visuals can sometimes be misleading. Even we, humans, might confuse a pile of weirdly-shaped snow for a dog or a mysterious silhouette, especially in the dark.[1]
ABI Research’s blog post, Multimodal learning and the future of artificial intelligence, outlined the impact and future of AI in business. Currently, AI devices work independently of one another, with high volumes of data flowing through each device. As AI continues developing, these devices will be able to powerfully work in accordance with one another, unveiling the full potential of AI, according to the post.[2]
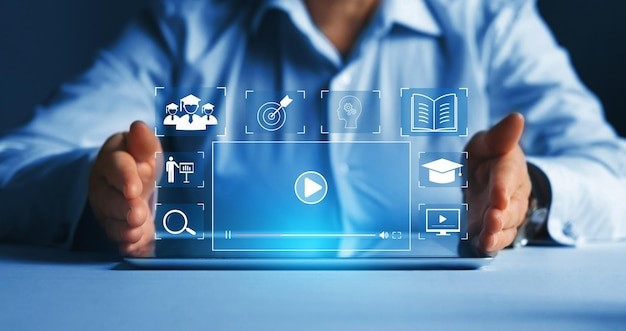
Figure .1 Multimodal learning
Figure 1 shows Multimodal learning is a type of machine learning that involves combining multiple modalities or types of data, such as text, images, audio, and video, to improve the accuracy of predictive models. The idea behind multimodal learning is that by leveraging information from multiple sources, a model can learn more robust and accurate representations of the underlying data.
Multimodal learning has applications in a wide range of fields, such as natural language processing, computer vision, and speech recognition. For example, in natural language processing, multimodal models can be used to generate more accurate and context-aware responses by combining information from text, audio, and video. In computer vision, multimodal models can be used to improve object recognition by incorporating information from different types of images, such as colour, grayscale, and depth.
One of the challenges of multimodal learning is how to effectively combine information from different modalities. Researchers have developed a variety of techniques for multimodal fusion, such as early fusion, where the features from each modality are combined at the input layer, and late fusion, where the features from each modality are combined at the output layer. Other techniques include cross-modal attention mechanisms, which allow the model to selectively attend to information from different modalities based on their relevance to the task.
Multimodal learning is an active area of research, and there are many exciting developments in this field. As more and more types of data become available, we can expect to see even more applications of multimodal learning in the future.
References:
- https://serokell.io/blog/multimodal-machine-learning
- https://www.techrepublic.com/article/multimodal-learning-the-future-of-artificial-intelligence/
Cite this article:
Janani R (2023), Multimodal learning, AnaTechMaz, pp.217