The Capabilities of Data Fabric
Gartner defines data fabric as a design concept that serves as an integrated layer (fabric) of data and connecting processes. A data fabric utilizes continuous analytics over existing, discoverable and inferenced metadata assets to support the design, deployment and utilization of integrated and reusable data across all environments, [1]including hybrid and multi-cloud platforms.
Data fabric leverages both human and machine capabilities to access data in place or support its consolidation where appropriate. It continuously identifies and connects data from disparate applications to discover unique, business-relevant relationships between the available data points. The insight supports re-engineered decision-making, providing more value through rapid access and comprehension than traditional data management practices. [1]
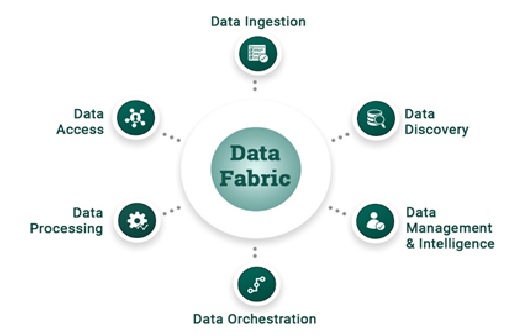
Figure 1. the capabilities of data fabric
Figure 1 shows Top performing enterprises are data driven. However, several challenges block them from fully exploiting all data. Lack of data access. Numerous data sources and data types.[2] Data integration complexities. Research shows that up to 74% of data is not analyzed in most organizations¹ and up to 82% of enterprises are inhibited by data silos².
With a data fabric, your business users and data scientists can access trusted data faster for their applications, analytics, AI and machine learning models, and business process automation, helping to improve decision making and drive digital transformation. [2]
Here are the steps to building a data fabric:
- Identify key sources of metadata
- Build a data model MVP
- Align data to the model
- Set up consumer applications
- Repeat for new data assets [4]
With AtScale’s A3 platform, Machine Learning (ML) delivers its autonomous capabilities with the goal to reduce human labor (or chores). The benefits deliver agile and governed analytics to the enterprise just-in-time, shifting resources from managing trillions of rows of data—to analyzing it.[3]
A true data fabric solution should have the following capabilities:
- Autonomous data engineering: Just-in-time query optimization for speed and usage consumption that can anticipate the needs of the data consumer in a single framework, reducing the complexity of data management and adapting to the needs of the data consumer.
- Unified data semantics: A workplace for all data for consumers to define business meaning and get a single-source-of-truth (SSOT) point regardless of structure, database technology, and deployment platform for a cohesive analytics experience.
- Centralized data security & governance: A centralized security policy to decentralize access using the tenets of Zero Trust that are applied consistently across the infrastructure for all data whether in cloud, multi-cloud, hybrid, or on-premises deployments.
- Data management visibility: The ability to measure data responsiveness, availability, reliability, and risk in a centralized workspace.
- Agnostic to platform and application: An ability to quickly integrate with data platform or BI/ML application for freedom of choice for data managers and consumers alike.
- Future-proofs infrastructure: Reduce the disruption of new technologies and data types while modernizing legacy systems to maximize investments. New infrastructure deployments are integrated without impact to existing infrastructure and deployments.
- No need for data movement: Intelligent data virtualization creates a single representation of data from multiple, disparate sources without having to copy or move the data.[3]
References:
- https://www.gartner.com/smarterwithgartner/data-fabric-architecture-is-key-to-modernizing-data-management-and-integration
- https://www.ibm.com/in-en/analytics/data-fabric
- https://www.atscale.com/blog/what-is-a-data-fabric/
- https://www.stardog.com/blog/5-steps-to-building-a-data-fabric/
Cite this article:
Thanusri swetha J (2021), The Capabilities of Data Fabric, Anatechmaz, pp. 23