Data Quality Management (DQM)
These days, data is the livelihood of any business. Data helps a company predict customer expectations, obtain competitor information, execute effective product management, and make informed top-down decisions. There is no doubt big data has a tremendous impact on the trajectory of any business.
The rise of the Data Quality Management (DQM) trend is a welcome relief for all businesses. Data quality management is an integral process that combines technology, process, the right people, and organizational culture to deliver data that is not only accurate but also useful. More importantly, data quality is not about being good or bad; its a range of metrics that measure the health of data used for analysis.
Data quality management (DQM) provides insights into data pumping through a business. It improves the data governance framework and enforces data standardization, ensuring that data used for analysis can provide a clear picture of the day-to-day business operations. As a result, business leaders can make accurate decisions that drive the business forward. [1]
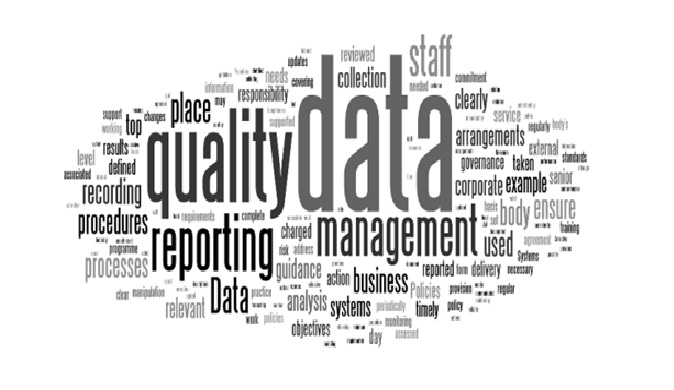
Figure 1. Data Quality Management (DQM)
Data Quality Management (DQM) is shown in figure 1. The proliferation of data in the digital age has presented a real challenge – data crisis. The data crisis entails low-quality data in its volumes that makes it hard for the businesses to make sense out of it, and in some instances, unusable. DQM has thereby come forth and has become an important process used to make sense out of data. It aims at helping organizations point out errors in their data that need to be resolved. It also aims at assessing if the data in their systems is accurate to serve the intended purpose.[2]
The 5 Pillars of Data Quality Management:
- The people
- Data profiling
- Defining data quality
- Data Reporting
- Data repair
Data Quality Management Best Practices:
- Ensure data governance
- Involve all departments
- Define a data glossary
- Find the root causes for quality issues
- nvest in automation
- Define DQM KPIs
- Integrate DQM and BI [3]
In conclusion, data quality management is essential for organizations to ensure the accuracy, completeness, consistency, and timeliness of their data. Poor data quality can lead to inaccurate analysis, misguided decision-making, and increased operational costs. Data quality management involves a series of activities, including data profiling, data cleansing, data enrichment, and data governance.
To achieve good data quality, organizations need to have a clear understanding of their data, establish data quality standards, implement data quality controls, and continuously monitor and improve the data quality. With effective data quality management, organizations can make informed decisions, improve customer satisfaction, increase operational efficiency, and gain a competitive advantage.
References:
- https://financesonline.com/business-intelligence-trends/
- https://www.dqlabs.ai/blog/what-is-data-quality-management/
- [3] https://www.datapine.com/blog/data-quality-management-and-metrics/#pillars
Cite this article:
Gokula Nandhini K (2023), Quantum Autonomous Transportation, Anatechmaz, pp.35