AntiFake's Innovative Approach to Defending Against Synthetic Speech Threats
In the rapidly evolving landscape of artificial intelligence, the strides made in generative technologies have given rise to both promising innovations and concerning challenges. One such challenge is the emergence of deepfakes, where synthesized speech can be manipulated to deceive humans and machines for malicious purposes. Addressing this growing threat, Ning Zhang, an assistant professor of computer science and engineering at the McKelvey School of Engineering at Washington University in St. Louis, has developed a groundbreaking tool called AntiFake. This tool takes a proactive approach, aiming to thwart unauthorized speech synthesis before it can be exploited.
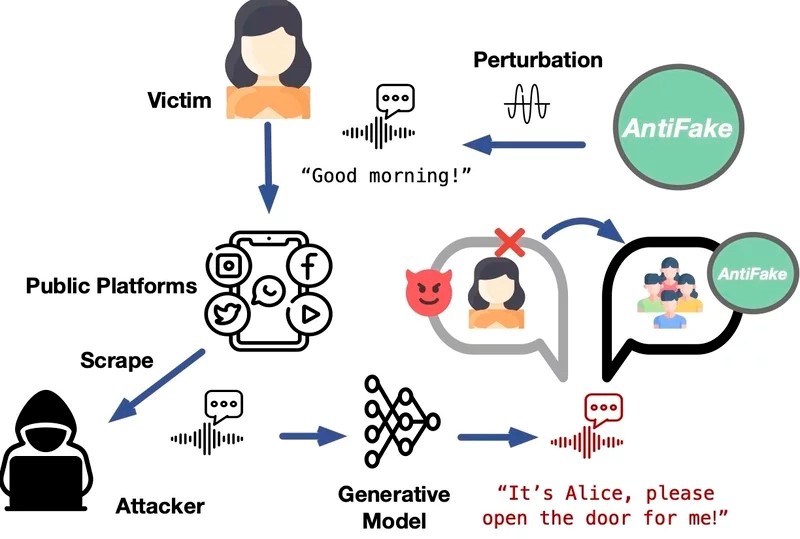
Figure 1. Overview of how AntiFake works. (Credit: Ning Zhang)
The Proactive Defense Mechanism
Figure 1 shows how AntiFake works. Presented at the Association for Computing Machinery's Conference on Computer and Communications Security in Copenhagen, AntiFake distinguishes itself from traditional deepfake detection methods. Instead of serving as a post-attack mitigation tool, it employs adversarial techniques to make the synthesis of deceptive speech more challenging. By disrupting AI tools' ability to extract necessary characteristics from voice recordings, AntiFake acts as a preemptive measure against potential misuse.
Adversarial AI in Action
The core of AntiFake lies in its use of adversarial AI, a technique originally found in the arsenal of cybercriminals. Zhang explains that the tool subtly distorts or perturbs recorded audio signals, making it difficult for criminals to use the information for voice synthesis and impersonation. Striking a delicate balance, AntiFake ensures that the altered audio remains perceptually accurate to human listeners while becoming unrecognizable to AI algorithms.
Free and Accessible
Emphasizing inclusivity, Zhang underscores that AntiFake's code is freely available to users. The tool's primary objective is to make it challenging for criminals to exploit voice data for deceptive purposes, thereby safeguarding individuals from potential harm.
Robust Testing and Results
To ensure AntiFake's effectiveness against a dynamic landscape of potential attackers and unknown synthesis models, Zhang and Zhiyuan Yu, the first author and a graduate student in Zhang's lab, designed the tool to be generalizable. Tested against five state-of-the-art speech synthesizers, AntiFake demonstrated a remarkable protection rate of over 95%, even when faced with unseen commercial synthesizers. Usability tests with 24 human participants confirmed that the tool is accessible to diverse populations.
Looking Ahead
While currently focused on protecting short clips of speech, AntiFake's adaptability holds promise for broader applications. Zhang envisions the tool expanding to safeguard longer recordings, and perhaps even music, in the ongoing battle against disinformation. As the field of AI voice technology continues to evolve, Zhang remains confident in AntiFake's strategy of turning adversaries' techniques against them, recognizing the enduring vulnerability of AI to adversarial perturbations.
In the quest to harness the benefits of AI, ensuring the responsible and secure development of these technologies is paramount. AntiFake stands as a beacon of innovation, offering a proactive defense against the misuse of synthetic speech. As we navigate an ever-changing technological landscape, tools like AntiFake play a crucial role in upholding authenticity and protecting individuals from the potential pitfalls of advanced AI capabilities.
Source: Washington University in St. Louis
Cite this article:
Hana M (2023), AntiFake's Innovative Approach to Defending Against Synthetic Speech Threats, AnaTechMaz, pp. 344