Unveiling the Secrets of Artificial Intelligence: Mimicking the Brain's Efficiency
In a groundbreaking study, Cambridge scientists have revealed that imposing physical constraints on artificial intelligence (AI) systems can lead to the development of brain-like features, mirroring the complex organization of the human brain. Drawing inspiration from the way biological brains navigate competing demands, the researchers applied constraints to their AI model, resulting in surprising similarities to the brains of living organisms.
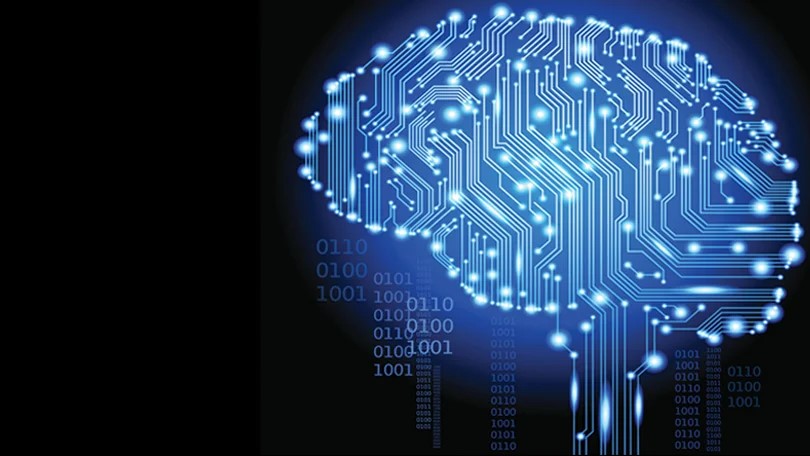
Figure 1. Illustration.
Understanding the Brain's Trade-offs
Figure 1 is an illustration. Neural systems in the brain face a constant trade-off between utilizing energy and resources to sustain the network physically while optimizing it for efficient information processing. This delicate balance, observed across species, often converges on similar organizational solutions. The researchers aimed to explore this phenomenon by creating an artificial system designed to simulate a simplified version of the brain.
Modeling the Brain
Instead of real neurons, the researchers utilized computational nodes in their AI system. These nodes functioned similarly to neurons, taking inputs, transforming them, and producing outputs. The key innovation was the introduction of a 'physical' constraint, assigning each node a specific location in a virtual space. The distance between nodes affected their ability to communicate, mirroring the organizational principles of the human brain.
Task and Learning
The researchers assigned the AI system a maze navigation task, akin to challenges presented to animals in neuroscience studies. The system, unaware of the solution initially, learned by adjusting the strength of connections between its nodes based on feedback. The physical constraint, reflecting the energy costs of long-distance connections in the human brain, prompted the emergence of hubs—highly connected nodes facilitating information flow.
Brain-Like Characteristics
Surprisingly, the AI system exhibited brain-like characteristics. It developed flexible coding schemes in individual nodes, allowing them to encode multiple properties of the maze simultaneously. This flexibility, seen in the brains of complex organisms, demonstrated the system's ability to adapt to constraints and find efficient solutions.
Implications for Understanding the Human Brain
The researchers believe that their AI system could provide insights into how constraints contribute to individual differences in human brains, shedding light on cognitive and mental health disparities. Artificial 'brains' offer a unique experimental platform to explore and understand the complexities of neural activity.
AI System as a Tool for Research
The artificial 'brains' developed by the team offer a tool for investigating neural activity and understanding the data recorded in real brains. This experimental approach enables researchers to explore questions that may be challenging to address in biological systems.
Future AI Systems and Design
The findings hold implications for the AI community, suggesting that physical constraints can contribute to the development of more efficient systems. The study proposes that, in situations with physical limitations, AI systems could benefit from architectures that resemble the structure and organization of the human brain.
As we delve deeper into the realms of artificial intelligence, understanding the intricate interplay between physical constraints and problem-solving capabilities becomes crucial. The research conducted by Cambridge scientists not only unveils the potential of AI systems to emulate brain-like features but also offers a glimpse into how future AI architectures could be designed for optimal efficiency. The quest to unlock the secrets of the human brain through artificial intelligence continues to open new doors to innovation and understanding.
Source: University of Cambridge
Cite this article:
Hana M (2023), Unveiling the Secrets of Artificial Intelligence: Mimicking the Brain's Efficiency, AnaTechMaz, pp. 336