How Crowdsourced Feedback is Transforming AI Training
In the fast-evolving realm of artificial intelligence (AI), researchers are constantly exploring innovative ways to enhance the learning capabilities of AI agents. One significant challenge in this process has been the time-consuming task of designing a reward function – an incentive mechanism crucial for guiding AI agents through the trial-and-error process of reinforcement learning.
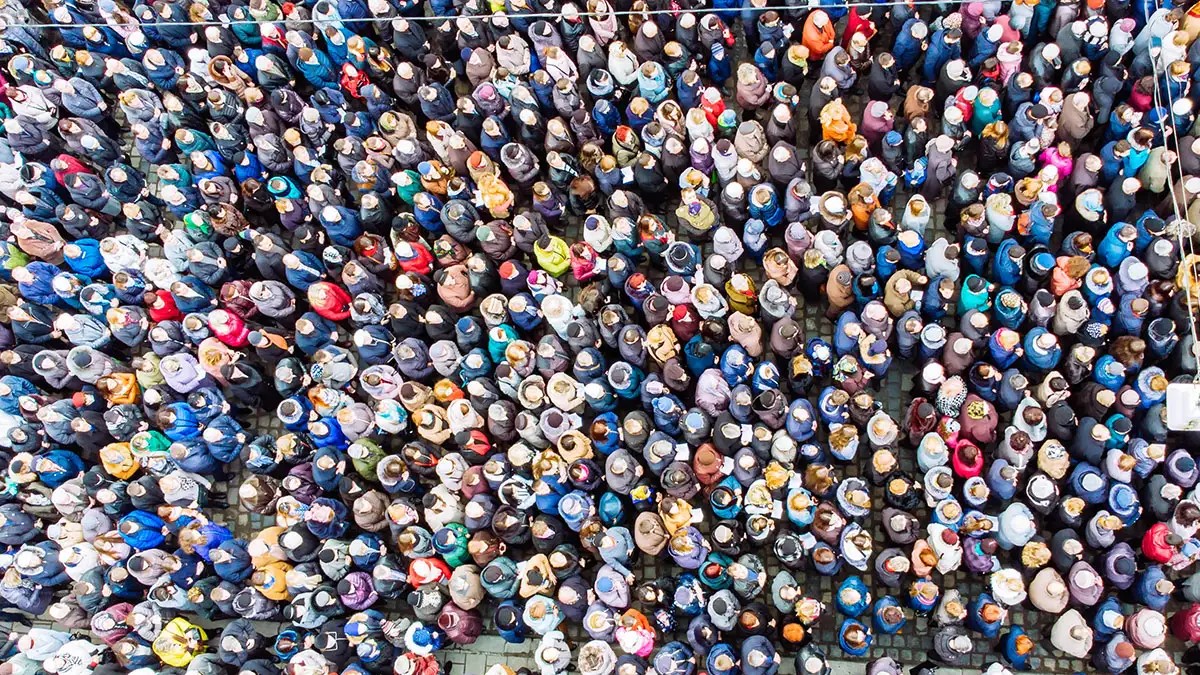
Figure 1. Crowd.
Figure 1 is an illustration. However, a recent breakthrough by researchers from MIT, Harvard University, and the University of Washington promises to revolutionize this landscape. They've developed a groundbreaking reinforcement learning approach that eliminates the need for a meticulously designed reward function. Instead, the method harnesses the power of crowdsourced feedback from nonexpert users to steer the AI agent toward its learning goals.
The Conundrum of Reward Functions
Traditionally, developing a reward function has been a bottleneck in the training of AI agents. Expert researchers painstakingly design these functions, which becomes a limiting factor when attempting to teach robots various complex tasks. The newly proposed approach not only bypasses this bottleneck but also introduces scalability by allowing nonexperts worldwide to contribute to the training process.
The Human-Guided Exploration (HuGE) Method
The HuGE method represents a departure from conventional reinforcement learning. It utilizes crowdsourced feedback, even if somewhat inaccurate, to guide the agent's exploration rather than constructing a rigid reward function. This approach cleverly incorporates asynchronous feedback, enabling nonexpert users globally to contribute to the agent's learning process.
How HuGE Works
The HuGE method operates through a two-pronged algorithmic approach. On one side, a goal selector algorithm continuously updates based on crowdsourced feedback, serving as a guide for the AI agent's exploration. On the other side, the AI agent autonomously explores, collecting data on its actions. This data is then sent to humans to update the goal selector, refining the agent's focus and accelerating its learning.
Advantages and Real-world Applications
Testing the HuGE method on both simulated and real-world tasks, researchers found that it outperformed other methods in terms of speed and efficiency. In real-world tests, robotic arms trained using HuGE learned tasks like drawing letters and object manipulation faster than alternative approaches.
The Future of HuGE
The researchers envision further refinements to the HuGE method, exploring its application in teaching multiple agents simultaneously. They are also keen on expanding its capabilities to incorporate natural language and physical interactions, pushing the boundaries of what AI agents can learn and achieve.
The implications of this research are significant, not only in terms of accelerating AI training but also in democratizing the process by involving nonexperts globally. As we move forward, innovations like HuGE could play a pivotal role in realizing the full potential of AI in a variety of applications, from household tasks to complex industrial processes.
Source: Massachusetts Institute of Technology
Cite this article:
Hana M (2023), How Crowdsourced Feedback is Transforming AI Training, AnaTechMaz, pp. 340