Utilising Machine Learning More Frequently in Bioprocessing
Barkha Singhal, an assistant professor at Gautam Buddha University, emphasizes the growing importance of computation, particularly artificial intelligence (AI) and machine learning (ML), in bioprocessing. Singhal highlights the significant demand for bioproducts, which drives the need for advanced computational technologies like ML and AI. These technologies play a crucial role in meeting the demand for bioproducts and can enhance productivity in a cost-effective manner, ultimately promoting the development of a biobased economy.
Singhal and her colleague highlighted the role of machine learning (ML) in the bioeconomy. They emphasized the urgency of transforming the linear economy's "take-make-dispose" concept to a circular economy that prioritizes reducing, reusing, and recycling for sustainability. Singhal stated that ML tools have the potential to significantly reduce time, enhance productivity, and promote cost-effectiveness in bioproducts. This, in turn, will play a crucial role in advancing the development of a biobased economy and fostering circularity in the economic model.
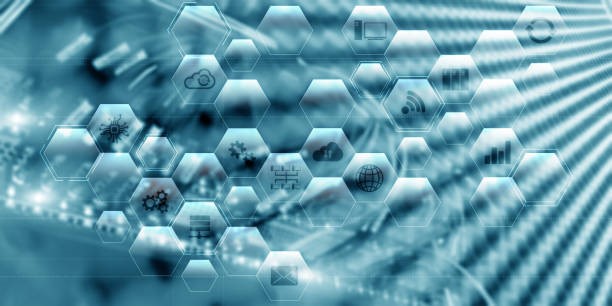
Figure .1 Utilising Machine Learning More Frequently in Bioprocessing
Figure 1 shows the digital transformation of bioprocesses from conventional to smart systems involves interconnectivity, real-time online monitoring, automation, artificial intelligence (AI), and machine learning (ML). The complexity and dynamic nature of bioprocesses, with numerous parameters and intricate interactions, pose challenges in controlling and maximizing bioproducts. Key factors, microbial communities, and metabolic interactions influence overall performance. Bioprocess digitization requires precise control techniques, including process classification, soft sensors, fault detection, and real-time monitoring. Data-driven predictive control and optimization are crucial for effectively operating bioprocesses, which are susceptible to operational changes. ML, along with image recognition, blockchain, and Internet of Things (IoT) technologies, plays a role in smart bioprocesses. ML-assisted circular bioeconomy is important for sustainable economic development. The special issue "Machine Learning for Smart Bioprocesses (MLSB-2022)" presents 23 manuscripts grouped into different categories, highlighting major findings.[1]
Fragments of sections
- Anaerobic digestion
- Thermochemical conversion
- Composting
- Microalgae identification
- Fungi bioprocessing
- Wastewater treatment
- Bioprocess optimization, monitoring, and control
- Biomolecule interactions
- echno-economic analysis and life cycle assessment [1]
Singhal highlights the broad applications of machine learning (ML) in improving various aspects of bioprocessing. ML can contribute to expanding the design space explored in bioprocesses and aid in the design of manufacturing facilities. It can be utilized in multiple facets of bioprocessing, including strain selection and engineering, optimization of bioprocessing, monitoring and control, and downstream processing. ML approaches have the potential to enhance these processes and improve overall efficiency in bioprocessing.
Increasing the use of ML in commercial bioprocessing depends on a variety of factors. "ML approaches will need to be implemented at the industry level," says Singhal. "Increased automation, data-driven development of bioprocesses, data availability, and exchange of data will be required." The transition that meets the need for timely production and paves the way for the prediction of bioproducts for futuristic applications is something that the present industries need to comprehend.[2]
In conclusion, the utilization of machine learning (ML) in bioprocessing is becoming increasingly prevalent and holds great potential for advancing the field. ML can play a significant role in various aspects of bioprocessing, including strain selection, process optimization, monitoring, and downstream processing. Its ability to analyse complex data, identify patterns, and make predictions can lead to improved efficiency, productivity, and cost-effectiveness in bioproduction. As the demand for bioproducts continues to rise, the integration of ML in bioprocessing workflows is essential for meeting these demands and promoting sustainability. With ongoing advancements in ML techniques and increased adoption in the industry, we can expect to see further advancements and innovations in bioprocessing in the coming years.
References:
- https://www.sciencedirect.com/science/article/abs/pii/S0960852423002523
- https://www.genengnews.com/topics/artificial-intelligence/making-more-use-of-machine-learning-in-bioprocessing/
Cite this article:
Janani R (2023),Utilising Machine Learning More Frequently in Bioprocessing, Anatechmaz, pp.292