Strong Protein Design Software Uses AI For Board Games
Scientists at the University of Washington have achieved a significant breakthrough in protein design by applying reinforcement learning, a strategy known for its success in board games like Chess and Go. The international research team developed a cutting-edge protein design software using this approach. In a notable experiment, proteins created with the new software demonstrated enhanced ability to generate valuable antibodies in mice. This achievement is considered a major milestone in leveraging artificial intelligence for protein science research. The implications of this development are extensive, ranging from the development of potent vaccines and improved cancer treatments to the creation of novel biodegradable textiles.
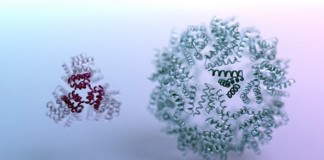
Figure .1 Strong Protein Design Software Uses AI For Board Games
Figure 1 shows Scientists led by David Baker at the University of Washington School of Medicine have successfully applied reinforcement learning, a strategy commonly used in board games, to protein science research. They developed a software that utilizes reinforcement learning to solve longstanding puzzles in protein science and create functional molecules. The software's ability to design complex protein nanomaterials with desired properties demonstrates the potential of reinforcement learning in protein design. The study, published in science, suggests that this approach could accelerate progress in various scientific fields.
The researchers emphasize the significance of multi-subunit protein assemblies in biology, which have evolved through natural selection to ensure optimal functionality. Naturally occurring protein assemblies exhibit shape complementarity among their subunits, resulting in architectures specifically suited for their intended functions. Current protein design methods struggle to achieve such optimization.
Traditionally, de novo protein design has followed a "bottom-up" hierarchical approach, starting with individual monomeric structures that assemble into larger oligomers to form the final protein assemblies. This approach has yielded designed protein assemblies with biomedical applications in fields like immunobiology, including the recent approval of a de novo-designed COVID vaccine. To overcome the limitations of the bottom-up protein complex design approach, David Baker's team developed a "top-down" methodology. This approach involves defining desired properties of the protein structure and systematically constructing subunits that optimize these properties when assembled.
The researchers utilized reinforcement learning (RL) and Monte Carlo tree search (MCTS) to create a program for protein design. The RL algorithm, implemented through MCTS, enabled the generation of protein complexes by directly optimizing global structural properties.The team trained the RL program by providing millions of starting molecules and allowing the software to make thousands of attempts to enhance each molecule towards a predefined goal. Through iterative processes, the program learned to manipulate the proteins into desired shapes.
The study focused on designing new nano-scale structures composed of multiple protein molecules. Electron microscopy experiments confirmed the successful formation of AI-designed nano-structures in the laboratory, demonstrating the potential of this approach for creating complex protein assemblies.
The team successfully synthesized and confirmed the realization of AI-designed proteins, including icosahedra and disk-shaped nanopores, in the laboratory. The structures closely matched the computational models and were distinct from existing ones. The software demonstrated high accuracy and customization, creating structures with different hole sizes. The atomically accurate design achieved by the software represents a significant advancement. The top-down reinforcement learning approach has the potential to overcome design challenges and enable the creation of therapeutic proteins and vaccines. The designed protein scaffolds showed improved performance in promoting blood vessel stability compared to previous versions.
The research conducted by the team has significant implications for regenerative medicine, as highlighted by co-author Hannele Ruohola-Baker. The increasing accuracy of the technology opens up possibilities for various applications in vascular treatments, such as addressing diabetes, brain injuries, strokes, and other conditions involving blood vessel risks. Moreover, it offers potential advancements in delivering differentiation factors to stem cells, providing new avenues for controlling cell development and aging.[1]
The authors emphasize that the MCTS approach's ability to optimize specified geometric criteria in a top-down manner paves the way for the development of potent, multivalent vaccines and cellular receptor agonists. These can be precisely designed to rigidly scaffold immunogen or receptor-binding monomers and position them accurately in relation to each other. This capability holds promise for enhancing vaccine effectiveness and creating targeted therapies.[1]
References:
- https://www.genengnews.com/news/powerful-protein-design-software-harnesses-board-game-playing-ai/
Cite this article:
Janani R (2023),Strong Protein Design Software Uses AI For Board Games, Anatechmaz, pp.287