Autonomous Vehicles Mastering Stabilize-Avoid Challenges with Machine Learning
In the thrilling movie "Top Gun: Maverick," Maverick, portrayed by Tom Cruise, leads a team of young pilots on a daring mission. They must skillfully navigate their jets through a treacherous canyon, flying at extremely low altitudes to avoid radar detection, and execute a steep climb while evading the canyon walls. Achieving this feat requires human intuition and expertise, but autonomous systems face significant challenges in handling such pulse-pounding tasks. The "stabilize-avoid problem" presents a conflict for AI-controlled aircraft, as the most direct path to the target conflicts with the need to avoid collisions and maintain stealth. Overcoming this problem is crucial for ensuring the safe achievement of mission objectives.
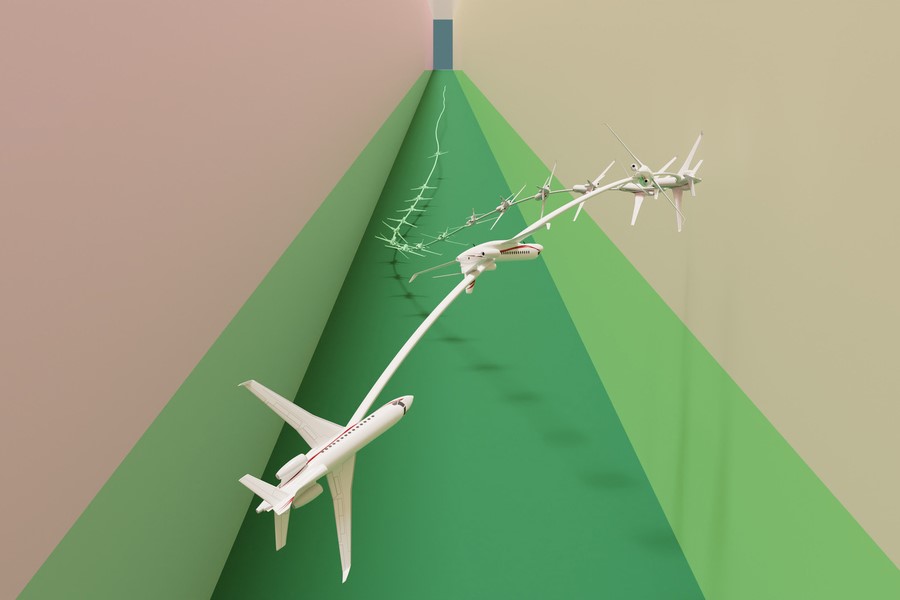
Figure 1. A simulated jet aircraft in a scenario where it had to stabilize to a target near the ground while maintaining a very low altitude and staying within a narrow flight corridor.
Figure 1 shows how the researchers used their technique to effectively fly a simulated jet aircraft in a scenario where it had to stabilize to a target near the ground while maintaining a very low altitude and staying within a narrow flight corridor. Addressing this longstanding challenge, MIT researchers have developed an innovative technique that outperforms existing methods in solving complex stabilize-avoid problems. Their machine-learning approach not only matches or surpasses the safety levels of current methods but also enhances stability by a factor of ten, enabling the agent to reach and maintain stability within the desired goal region.
In a remarkable simulation, the researchers successfully piloted a virtual jet aircraft through a narrow corridor without crashing into the ground. Traditional approaches often simplify the system, leading to inadequate results when faced with real-world dynamics. While reinforcement learning offers a more effective solution, balancing the dual goals of stability and obstacle avoidance remains tedious.
The MIT team tackled the problem by breaking it down into two steps. First, they reformulated the stabilize-avoid problem as a constrained optimization problem, with the optimization enabling the agent to reach and stabilize within the goal region. By applying constraints, the researchers ensured that the agent could successfully avoid obstacles. In the second step, they transformed the constrained optimization problem into the epigraph form and utilized a deep reinforcement learning algorithm to solve it. This mathematical representation allowed them to overcome the challenges faced by other reinforcement learning methods.
Their approach was rigorously tested through a series of control experiments with varying initial conditions. The agent had to stabilize within a goal region while executing evasive maneuvers to avoid oncoming obstacles. When compared to several baselines, the MIT technique stood out as the only one capable of stabilizing all trajectories while maintaining safety. In a captivating scenario reminiscent of a "Top Gun" movie, the researchers used their method to fly a simulated jet aircraft. The jet had to stabilize near the ground, maintaining a low altitude within a narrow flight corridor. Even in this complex setting, their controller outperformed all the baselines, preventing crashes and stalling.
Looking ahead, this groundbreaking technique holds promise for designing controllers for highly dynamic robots that require safety and stability, such as autonomous delivery drones. It could also be integrated into larger systems, aiding drivers in regaining control during skids on snowy roads, ensuring a safe return to a stable trajectory.
The MIT researchers envision reinforcing the technique to account for uncertainties in optimization and examining its performance when deployed on real-world hardware. By providing safety and stability guarantees, this research takes a promising first step toward deploying reinforcement learning controllers on mission-critical systems. Driven by their determination to navigate extreme scenarios surpassing human capabilities, the researchers aim to make significant advancements in the field and offer assurance in critical deployments.
Source: MIT
Cite this article:
Hana M (2023), Autonomous Vehicles Mastering Stabilize-Avoid Challenges with Machine Learning, AnaTechMaz, pp.314