A Chatgpt-Like Model That "Speaks Protein" To Hasten Drug Discovery
Conventional AI systems for drug screening are slow as they rely on calculating the three-dimensional structures of target proteins and predicting their interactions with drug molecules. To address this, researchers from MIT and Tufts University have created an alternative approach using a large language model called ConPLex. This model, similar to ChatGPT, can analyze vast amounts of text and determine the likelihood of specific amino acids appearing together. It enables matching target proteins with potential drug molecules without the need for computationally intensive structure calculations.
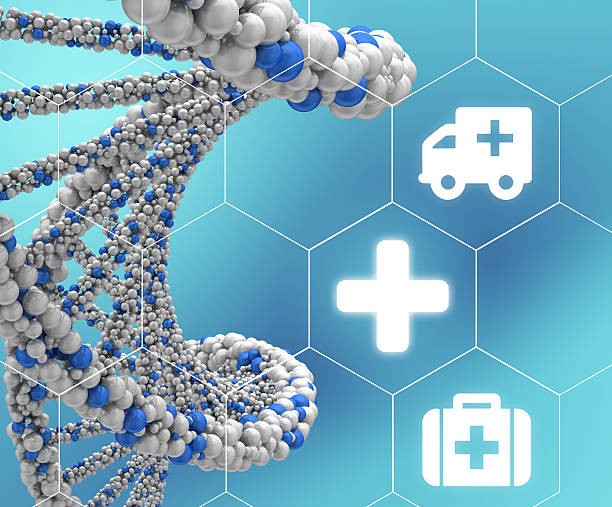
Figure 1. Running a drug screening program is comparable to monitoring a large cocktail party, where most conversations are trivial but a few are significant. Similarly, in drug screening, there are numerous weak drug-target interactions compared to high-affinity binding events. Evaluating every interaction is tedious and exhausting for AI systems.
The researchers developed ConPLex, a computational approach that combines pretrained protein language models (PLex) and protein-anchored contrastive coembedding (Con). This approach outperforms existing methods in terms of accuracy, adaptivity to new data, and specificity against decoy compounds. ConPLex enables predictions of binding by utilizing the distance between learned representations, making it capable of screening massive compound libraries and the human proteome. The model was tested by screening around 4,700 drug candidate molecules for their binding potential to a set of 51 protein kinases. Figure 1 shows AI systems.
After testing the top hits from ConPLex, the researchers selected 19 drug-protein pairs for experimental testing. The results demonstrated that 12 out of the 19 pairs exhibited strong binding affinity in the nanomolar range, while the majority of other drug-protein combinations showed no affinity. Notably, four of these pairs demonstrated exceptionally high sub-nanomolar affinity, where minute drug concentrations in parts per billion inhibited the protein effectively.
While the study primarily focused on small-molecule drugs, the researchers are now exploring the application of this approach to other drug types, including therapeutic antibodies. Furthermore, this modeling technique holds potential for conducting toxicity screenings to ensure the absence of unwanted side effects in potential drug compounds before animal testing.
Bonnie Berger, a senior author of the study and an MIT researcher, highlighted the significance of this work in meeting the demand for efficient and accurate in silico screening of potential drug candidates. The developed model, ConPLex, facilitates large-scale screening to assess off-target effects, explore drug repurposing opportunities, and evaluate the impact of mutations on drug binding. This approach opens up possibilities for accelerating drug discovery and development processes.
Rohit Singh, a researcher at MIT and one of the study's primary authors, observed that "the high failure rates of drug discovery are part of the reason why it is so expensive." The expense of drug discovery could be significantly decreased if we can lessen those failure percentages by acknowledging up front that this medicine is unlikely to be successful.[1]
References:
- https://www.genengnews.com/topics/artificial-intelligence/chatgpt-like-model-speaks-protein-to-speed-drug-discovery/
Cite this article:
Janani R (2023), A Chatgpt-Like Model That "Speaks Protein" To Hasten Drug Discovery, AnaTechMaz. pp.308