Brain-Inspired Computing: UD Physicist Explores Low-Energy Solutions with Nanomagnets
The human brain is an incredible organ, inspiring scientists across fields — including physicists, engineers, and computer scientists — to study its unique methods of storing, processing, and utilizing information. Among these researchers is Benjamin Jungfleisch, associate professor of physics at the University of Delaware, whose expertise in magnon spintronics could revolutionize energy-efficient computing.
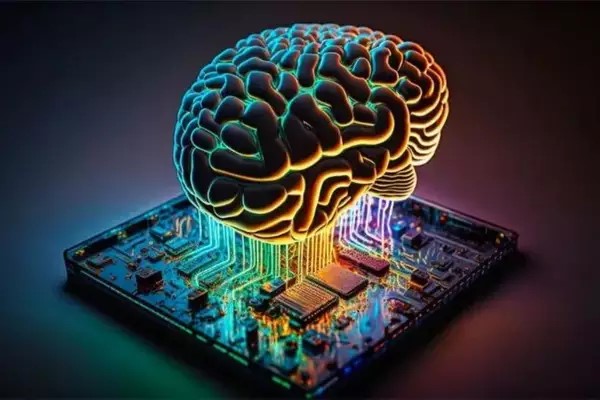
Figure 1. Brain-Inspired Computing.
Joining UD’s faculty in 2018, Jungfleisch specializes in using lasers to examine the dynamics of magnetic nanostructures, or tiny magnets that store and transmit information through circuits. His current focus is on creating brain-inspired, low-power computing solutions by leveraging interacting nanomagnets as an information-processing center. Figure 1 is an illustration of brain-inspired computing.
In much the same way neurons transfer information in the brain, magnons — quantum excitations in magnetic systems that generate "spin waves" — carry and process data within magnetic nanostructure networks. This mimics the brain’s energy-efficient processing capabilities and holds promise for AI and other high-demand applications, where energy consumption has become a growing concern. Without such innovations, the computational demand of AI will continue to require increasingly large data centers, posing environmental challenges.
The National Science Foundation recently awarded Jungfleisch a 2024 CAREER Award, a five-year grant totaling over $798,000, to further this research. With support from the EPSCoR program, Jungfleisch’s work focuses on advancing low-power computing via four key research areas:
- Controlling magnons in two-dimensional nanomagnetic arrays
- Manipulating interactions between magnons
- Deepening the understanding of magnetic nanostructure dynamics
- Experimentally demonstrating neuromorphic (brain-like) processing concepts [1]
Recent publications in Nature Communications by Jungfleisch and collaborators reveal advances in magnon-magnon coupling and nonlinear dynamics. Notably, his work is progressing toward three-dimensional nanomagnetic structures, which offer increased performance, more storage capacity, and reconfigurability. These 3D networks also present exciting nonlinear dynamics: “one magnon can split into two, or two magnons can merge into one.” This property enables parallel computing and complex information processing.
A breakthrough in 2022 by Jungfleisch’s collaborator, physicist Jack Gartside of Imperial College London, builds on Jungfleisch’s previous discoveries and demonstrates that these nanomagnetic networks could be trained for predictive tasks. Future systems could complete training cycles in minutes instead of hours by leveraging phenomena like spin torque.
Jungfleisch’s research will also address remaining questions, including how to introduce and control randomness within these systems. During his upcoming sabbatical, he plans to collaborate with research groups in Germany and India to further refine these concepts.
Additionally, Jungfleisch’s CAREER project includes a public outreach component. He aims to develop a five-week magnetism and electricity course for UD’s Osher Lifelong Learning Institute, as well as create a hands-on wave machine demonstration using accessible materials, such as barbeque skewers, duct tape, and gummy bears. This device will be loaned to local schools to support STEM education.
Comparing these nanomagnetic arrays to the brain’s neural networks, Jungfleisch explained: “These arrays of interacting nanomagnets are essentially just tiny bar magnets… they can store information very similar to what the neurons do in our brain.”
Traditional computing requires constant data transfer between separate processing and memory units, creating inefficiencies. But as Jungfleisch pointed out, interacting nanomagnet devices eliminate this issue by performing both processing and storage within the same unit. “These structures can do it all,” he said. “We do not need electrons, because we use magnetic excitations. And second, we can do processing and storage at the same time in the same unit.”
As Jungfleisch continues his work, he hopes that nanomagnet networks may soon perform complex AI tasks — from image generation to text-based interactions, like those offered by ChatGPT.
Source: University of Delaware
References:
- https://www.eurekalert.org/news-releases/1063959
Cite this article:
Hana M (2024), Brain-Inspired Computing: UD Physicist Explores Low-Energy Solutions with Nanomagnets, AnaTechMaz, pp. 315