Advancements in Analog Learning Systems: Scaling Complexity and Efficiency
Scientists encounter numerous tradeoffs when developing and expanding brain-like systems capable of performing machine learning. For example, while artificial neural networks excel at learning complex tasks in language and vision, the training process is slow and demands substantial power.
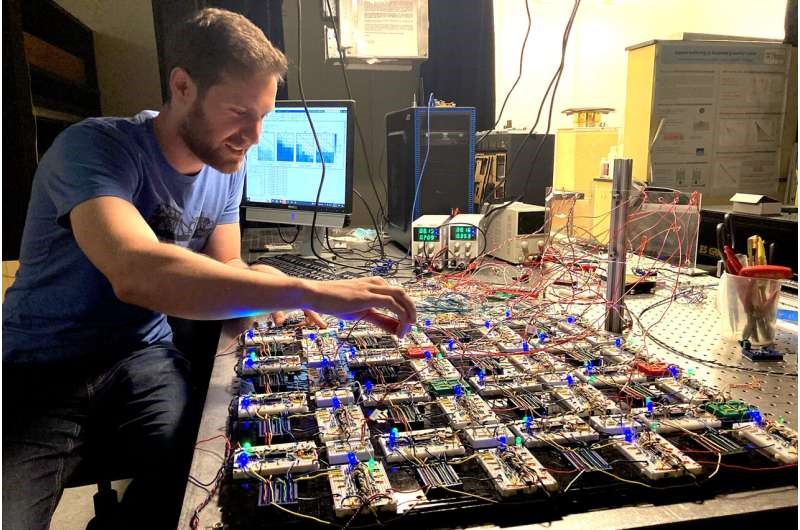
Figure 1. The Proposed Method. (Credit: Erica Moser)
Figure 1 shows Sam Dillavou, a postdoc in the Durian Research Group in the School of Arts & Sciences, built the components of this contrastive local learning network, an analog system that is fast, low-power, scalable, and able to learn nonlinear tasks.
To mitigate these challenges, researchers explore digital training with analog task execution, where input varies based on physical quantities like voltage. Although this approach reduces time and power consumption, small errors can quickly accumulate. Previously designed electrical networks, however, offer scalability without compounding errors, but are limited to linear tasks with straightforward input-output relationships [3].
Now, researchers have introduced an analog system that is fast, energy-efficient, scalable, and capable of learning more complex tasks such as "exclusive or" relationships and nonlinear regression. Known as a contrastive local learning network, this system evolves its components independently based on local rules, analogous to neurons in the human brain where learning emerges without global awareness.
[1] Physicist Sam Dillavou describes the system as a physical object that can learn to perform tasks similarly to computational neural networks. "One of the things we’re really excited about is that, because it has no knowledge of the structure of the network, it’s very tolerant to errors, it’s very robust to being made in different ways, and we think that opens up a lot of opportunities to scale these things up," says engineering professor Marc Z. Miskin.
"I think it is an ideal model system that we can study to get insight into all kinds of problems, including biological problems," adds physics professor Andrea J. Liu. She also suggests potential applications in interfacing with data-collecting devices such as cameras and microphones [2].
The researchers emphasize that their self-learning system "provides a unique opportunity for studying emergent learning. In comparison to biological systems, including the brain, our system relies on simpler, well-understood dynamics, is precisely trainable, and uses simple modular components."
The framework, termed Coupled Learning, was devised by Liu and postdoc Menachem (Nachi) Stern and published in 2021. This approach allows a physical system to adapt to tasks through applied inputs using local learning rules and no centralized processor.
Dillavou, who focused on translating the framework into its current physical design at Penn, notes, "One of the craziest parts about this is the thing really is learning on its own; we’re just kind of setting it up to go." Researchers input voltages, and the system's transistors update their properties based on Coupled Learning rules..
"Because the way that it both calculates and learns is based on physics, it’s way more interpretable," explains Miskin. "You can actually figure out what it’s trying to do because you have a good handle on the underlying mechanism."
Durian hopes this research marks "the beginning of an enormous field," highlighting ongoing efforts to scale up the design and explore fundamental questions about memory storage, noise effects, network architecture, and nonlinearities.
Miskin concludes, "If you think of a brain, there’s a huge gap between a worm with 300 neurons and a human being, and it’s not obvious where those capabilities emerge, how things change as you scale up. Having a physical system which you can make bigger and bigger is an opportunity to actually study that."
Source: University of Pennsylvania
References:
- https://techxplore.com/news/2024-07-physical-nonlinear-tasks-traditional-processor.html
- https://www.eurekalert.org/news-releases/1050612
- https://blog.seas.upenn.edu/a-first-physical-system-learns-nonlinear-tasks-without-a-traditional-computer-processor/
Cite this article:
Hana M (2024), Advancements in Analog Learning Systems: Scaling Complexity and Efficiency, AnaTechMaz, pp. 310