Statistical Foundation for Data Science
Statistical Foundations of Data Science gives a thorough introduction to commonly used statistical models, contemporary statistical machine learning techniques and algorithms, along with their mathematical insights and statistical theories.
It aims to serve as a graduate-level textbook and a research monograph on high-dimensional statistics, sparsity and covariance learning, machine learning, and statistical inference. It includes ample exercises that involve both theoretical studies as well as empirical applications. [1]
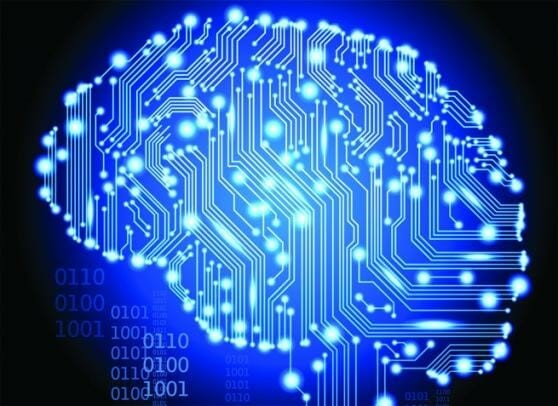
Figure 1. Statistical Foundation for Data Science
Statistical Foundation for Data Science is shown in figure 1. The statistical foundation is an essential component of data science, providing the theoretical framework and tools for analyzing and interpreting data. Here are some key statistical concepts that form the foundation for data science:
- Descriptive Statistics
- Probability Theory
- Statistical Inference
- Regression Analysis
- Experimental Design
- Statistical Learning
- Bayesian Statistics
- Time Series Analysis
These are just some of the key statistical concepts that provide the foundation for data science. A solid understanding of these concepts enables data scientists to effectively analyze data, draw meaningful insights, and make informed decisions based on data-driven evidence.
Types of statistics:
- Descriptive statistics: It assists in the organization of data and focuses on the data’s most important properties. It presents a numerical or graphical overview of the data. Numerical metrics such as average, mode, standard deviation, or SD, as well as correlation, are used to explain the data set’s characteristics.
- Inferential statistics: It uses probability theory to generalize the more extensive data set. It allows you to model relationships within the data and deduce population parameters based on sample statistics. You can use modeling to create mathematical equations that describe the relationships between two or more variables.
Importance of statistics in data science
Data is ingrained in today’s world; individuals and businesses generate vast amounts of data that professionals can only view and comprehend. While a career in data science may appear appealing and accessible, aspiring Data Scientists should assess their familiarity with statistics before making their next move. Statistics provides the techniques and tools for discovering structure in large datasets and provides individuals and organizations with a better awareness of the realities revealed by their data.[2]
References:
- https://www.routledge.com/Statistical-Foundations-of-Data-Science/Fan-Li-Zhang-Zou/p/book/9781466510845
- https://www.infosectrain.com/blog/how-to-build-your-statistical-foundations-for-a-career-in-data-science/
Cite this article:
Gokula Nandhini K (2023), Statistical Foundation for Data Science, Anatechmaz, pp.88