GIS and spatial data
Spatial data can be referred to as geographic data or geospatial data. Spatial data provides the information that identifies the location of features and boundaries on Earth. Spatial data can be processed and analysed using Geographical Information Systems (GIS) or Image Processing packages.
Types of data
There are different types of spatial data which can be split into two categories:
- Feature Data (vector data model) = entity of the real world e.g. a road, a tree or a building these can be represented as a point, line or polygon in space.
- Coverage Data (raster data model) = mapping of continuous data in space expressed as a range of values e.g. a satellite image, an aerial photograph, a Digital Surface Model (DSM) or Digital Terrain Model (DTM), text file with daily precipitation values. Coverage data can be represented as a grid or triangulated irregular network. [1]
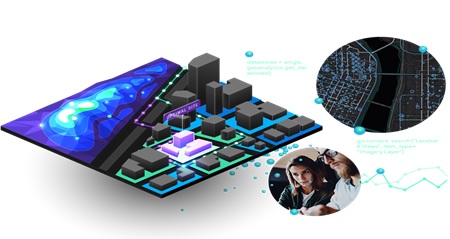
Figure 1. GIS and spatial datas
GIS and spatial data is shown in figure 1. GIS can be a powerful tool for data scientists, who can use it to analyze and visualize data in a variety of ways.
In many cases, one of your first tasks as a newly-hired data scientist will be creating a data set to visualize, analyze, and solve a problem, so focus on developing your problem solving skills.[2]
Benefits of GIS and spatial data:
Geographic Information Systems (GIS) and spatial data play an important role in data science by providing a powerful set of tools and techniques for analyzing, visualizing, and interpreting data in a geospatial context. Here are some benefits of using GIS and spatial data in data science:
- Better understanding of spatial relationships
- Enhanced data visualization
- Improved decision-making
- Increased efficiency
- Better prediction and forecasting
GIS (Geographic Information System) and spatial data are essential components in data science, particularly in areas such as environmental science, urban planning, transportation, and public health. Overall, GIS and spatial data are important components of data science that can provide valuable insights and context. Their effective use requires specialized skills, knowledge, and software tools.
References:
- https://www.methods.manchester.ac.uk/themes/spatial-and-gis-methods/spatial-data/
- https://www.directionsmag.com/article/11693
Cite this article:
Gokula Nandhini K (2023), GIS and spatial data, Anatechmaz, pp.78