Smoothing Methods
Data smoothing is done by using an algorithm to remove noise from a data set. This allows important patterns to more clearly stand out.
Data smoothing can be used to help predict trends, such as those found in securities prices, as well as in economic analysis.[1]
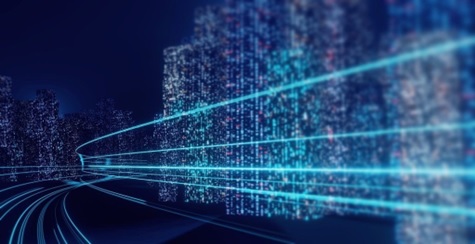
Figure 1. Smoothing methods
Smoothing methods is shown in figure 1. The random method, simple moving average, random walk, simple exponential, and exponential moving average are some of the methods used for data smoothing. Data smoothing can help in identifying trends in businesses, financial securities, and the economy.
Data Smoothing Methods
The random method, simple moving average, random walk, simple exponential, and exponential moving average are some of the methods that can be used for data smoothing.
1. Simple Exponential
The simple exponential method is a popular data smoothing method because of the ease of calculation, flexibility, and good performance. It uses an average calculation for assigning the exponentially declining weights beginning with the most recent observation. The method can be easily learned and applied.
The predictions are considered accurate since the difference between the real projections and what really happens is accounted for in the simple exponential approach. However, the method is not capable of managing trends well. Hence, it is used when cyclical variations are not present.
2. Moving Average
The moving average approach is best used when there is slight or no seasonal variation. Moving average data smoothing is used for separating random variation.
It is a simple data smoothing approach that economists use to help assess the underlying patterns in building permits and other volatile datasets. Moving average consolidates the month-long data points into time units longer than a month, such as an average of data of several months.
3. Random Walk
The random walk data smoothing method is commonly used for describing the patterns in financial instruments. Some investors think that the past movement in the price of a security and the future movements cannot be related. They use the random walk method, which assumes that a random variable will give the potential data points when added to the last accessible data point.
4. Exponential Moving Average
In the exponential moving average approach, weights are applied to historical observations after using the exponential smoothing method. It focuses more on the latest data observations. Hence, the exponential moving average responds faster to price changes than the simple moving average method.
Furthermore, the predictions only need the previous volatility prediction and the previous cycle price shift. The forecast is not needed to be recalculated using a long price returns history. [2]
Overall, smoothing methods are a valuable tool in the data scientist's toolbox, but they should be used judiciously and in combination with other analytical techniques to obtain a comprehensive understanding of the data.
References:
- https://www.investopedia.com/terms/d/data-smoothing.asp
- https://corporatefinanceinstitute.com/resources/business-intelligence/data-smoothing/
Cite this article:
Gokula Nandhini K (2023), Smoothing Methods, AnaTechMaz, pp.76