How AI Is Preserving the Mona Lisa: A Revolution in Digital Forensics
In the digital era, recovering deleted data poses a major challenge for digital forensics. As data volumes and storage methods grow, traditional recovery techniques are becoming less effective. The Carve-DL research project addresses this issue with an AI-driven solution that enhances the efficiency and accuracy of digital data reconstruction using advanced learning algorithms.
Conventional forensic data recovery relies on standardized, often manual methods based on fixed file signatures or file system metadata. Carve-DL revolutionizes this process with advanced deep learning technologies, including Swin Transformer V2 and ResNet. This AI-driven approach enables the recovery of complete files and the reconstruction of highly fragmented data, achieving precision where traditional techniques fall short.
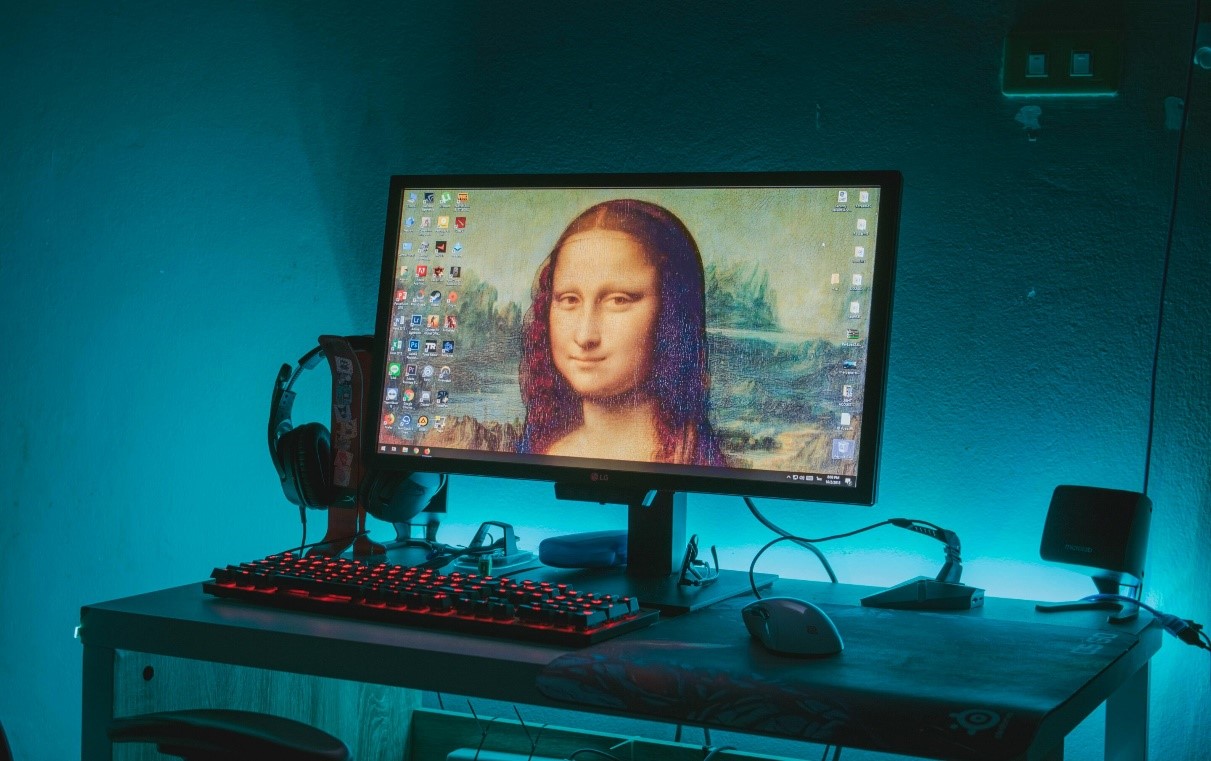
Figure 1. AI's Role in Digital Forensics Transformation
Carve-DL is designed for digital forensics specialists who need to recover deleted or fragmented data. It enables the reconstruction of automatically deleted website cache data relevant to investigations and helps restore manipulated or intentionally destroyed digital evidence using AI. Figure 1 shows AI's Role in Digital Forensics Transformation.
Case Study: Unraveling the Mona Lisa's Disappearance
The video accompanying this case study presents a fictional crime story to demonstrate how Carve-DL can recover deleted image data. In the scenario, the Mona Lisa is stolen, and all digital traces of the crime are erased. The video shows how Carve-DL reconstructs the original image of the stolen painting from fragmented memory data found on the thief's device, allowing for forensic analysis. This example highlights the practical advantages of the AI system: it can identify, classify, group, and correctly arrange deleted image fragments—capabilities that are essential for real-life digital evidence recovery. The full video is available in the attachment to this news.
- Classification Model: Enhanced identification of file types in raw data to improve recovery efficiency.
- Verification Model: Specialized model ensuring accurate reconstruction of image fragments.
- Clustering Techniques: Deep learning-based clustering methods effectively group related file fragments.
- Reordering Model: Advanced fragment reordering achieving a 95% accuracy rate in assembling reconstructed images.
Key Technological Milestones
Since the project's launch in November 2022, significant advancements have been made in optimizing the AI workflow for digital forensics and data reconstruction:
The integration of Swin Transformer V2 and ResNet has notably improved system performance. Additionally, Supportive Clustering with Contrastive Learning (SCCL) has boosted clustering accuracy to approximately 85%.
Challenges and Cutting-Edge Solutions
A major challenge in the project was the unpredictable number and nature of fragments to be reconstructed. Carve-DL tackled this by incorporating iterative clustering early in the pipeline to manage this uncertainty effectively. Additionally, scalable and efficient fragment reordering posed a difficulty [1]. To overcome this, the team integrated digital signal processing with low-rank approximation (LoRA), optimizing computing resource efficiency.
Applications Beyond Forensics
Beyond police investigations, Carve-DL offers promising applications in various fields:
- Data recovery in industries, such as restoring lost research data.
- Digital restoration and archiving for preserving historical documents.
- Cybersecurity, aiding in the analysis of data manipulation or targeted deletions.
As the Carve-DL project approaches its conclusion in October 2025, researchers highlight its success. The AI-driven techniques developed have the potential to revolutionize digital forensics, enabling highly precise recovery of deleted or fragmented data.
Reference:
- https://techxplore.com/news/2025-03-ai-mona-lisa-paradigm-shift.html
Cite this article:
Janani R (2025), How AI Is Preserving the Mona Lisa: A Revolution in Digital Forensics, AnaTechMaz, pp. 596