OpenAI Unveils GPT-4o Image Generation: Features, Availability, and More
New research from MIT and NVIDIA could enable real-time corrections of a robot’s actions through intuitive feedback, similar to how you would guide another person. For instance, if a robot misses grabbing a soapy bowl from the sink, you could easily correct it by pointing to the bowl, tracing its path on a screen, or gently nudging the robot's arm to steer it in the right direction.
Unlike other approaches that require data collection and retraining, this new technique allows robots to adapt through intuitive, real-time feedback from users. It helps the robot select an optimal action sequence based on the user's intent. In tests, the framework outperformed an alternative method by 21 percent. Over time, this could allow users to easily guide a factory-trained robot to perform various household tasks, even if the robot has never encountered their home or its objects.
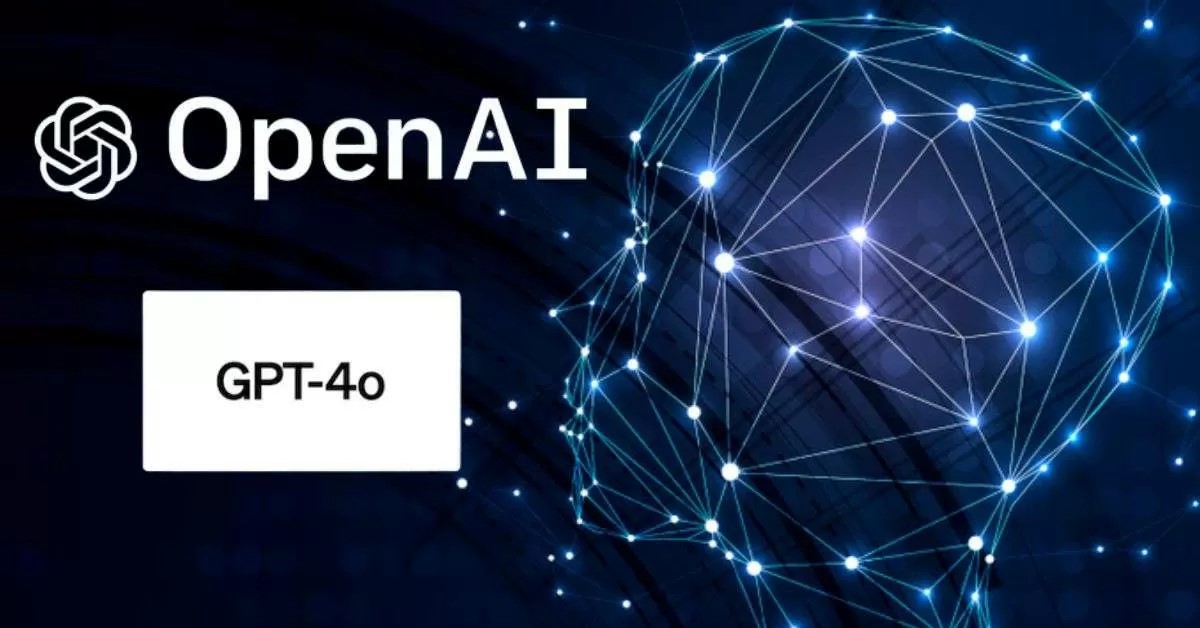
Figure 1. OpenAI Launches GPT-4o Image Generation: Key Features & Availability
Felix Yanwei Wang, the lead author of the paper and an EECS graduate student, emphasizes that consumers expect robots to work immediately out of the box, without the need for data collection or fine-tuning. His team addressed this challenge by developing an intuitive customization method for robots [1]. Co-authors include Lirui Wang, Yilun Du, senior author Julie Shah, and others from MIT and NVIDIA. The research will be presented at the International Conference on Robots and Automation. Figure 1 shows OpenAI Launches GPT-4o Image Generation: Key Features & Availability.
The research focuses on addressing misalignment issues in artificial intelligence. It aims to develop methods for ensuring AI systems align with human values and objectives, minimizing risks and improving reliability. By exploring strategies to manage and correct misalignment, the work seeks to enhance the safety and effectiveness of AI in various applications.
Researchers are exploring how pre-trained generative AI models can help robots learn a "policy" for completing tasks by generating valid motion trajectories. While these trajectories are feasible, they may not always align with the user's intent in real-world scenarios, especially if the robot encounters unfamiliar conditions. Traditionally, engineers would collect new data and retrain the model to handle such cases, but this process is time-consuming and requires expertise. MIT researchers aim to enable users to correct the robot's actions in real-time, but this could inadvertently lead to the generative model selecting invalid actions, such as completing the task but causing unintended consequences.
The researchers developed a framework that lets users correct a robot’s behavior without introducing errors, ensuring the robot’s actions align with the user’s intent while remaining feasible. The framework provides three intuitive methods for users to guide the robot: pointing to the object on an interface showing the robot's camera view, tracing a desired trajectory, or physically nudging the robot's arm to direct its movement. Wang emphasizes that physically nudging the robot is the most effective way to communicate user intent, as it avoids the loss of information that can occur when mapping a 2D image to 3D actions.
Sampling for Optimal Outcomes
To ensure that user interactions do not lead to invalid actions, such as object collisions, the researchers implemented a specific sampling procedure. This technique allows the robot to select actions that closely align with the user’s goal while remaining within its learned behaviors.
“Instead of simply following the user’s commands, the robot uses the sampling process to balance the user’s intent with its learned actions,” Wang explains.
This method proved more effective than alternative approaches during simulations and experiments with a real robot arm in a toy kitchen.
Although the method may not always complete a task immediately, it allows users to quickly correct the robot's mistakes in real-time, rather than waiting for it to finish before issuing new instructions [2]. Additionally, after several corrective nudges, the robot can log the action and incorporate it into future tasks, eliminating the need for further corrections.
“The key to continuous improvement is allowing the user to interact with the robot, which we’ve demonstrated here,” Wang says.
Looking ahead, the researchers aim to enhance the speed of the sampling procedure without sacrificing performance and explore robot policy generation in new environments.
Reference:
- https://www.digit.in/news/general/openai-launches-gpt4o-image-generation-availability-features-and-more.html
- https://www.theverge.com/openai/635118/chatgpt-sora-ai-image-generation-chatgpt
Cite this article:
Janani R (2025), OpenAI Unveils GPT-4o Image Generation: Features, Availability, and More, AnaTechMaz, pp. 588