AI Breakthrough Uncovers Hidden Signals in the Universe
A machine learning tool aims to unlock fundamental insights about the universe.
A new AI-powered tool enables scientists to process vast LIGO data autonomously. It detects environmental noise sources, including earthquakes and ocean waves, that disrupt gravitational wave signals, enhancing detection accuracy.
Leveraging Machine Learning for Gravitational Wave Analysis
Researchers at the University of California, Riverside, have developed a machine learning tool to identify patterns and reduce noise in LIGO’s gravitational wave data. Presented at an IEEE big-data workshop, their unsupervised approach could also benefit particle accelerators and industrial systems.
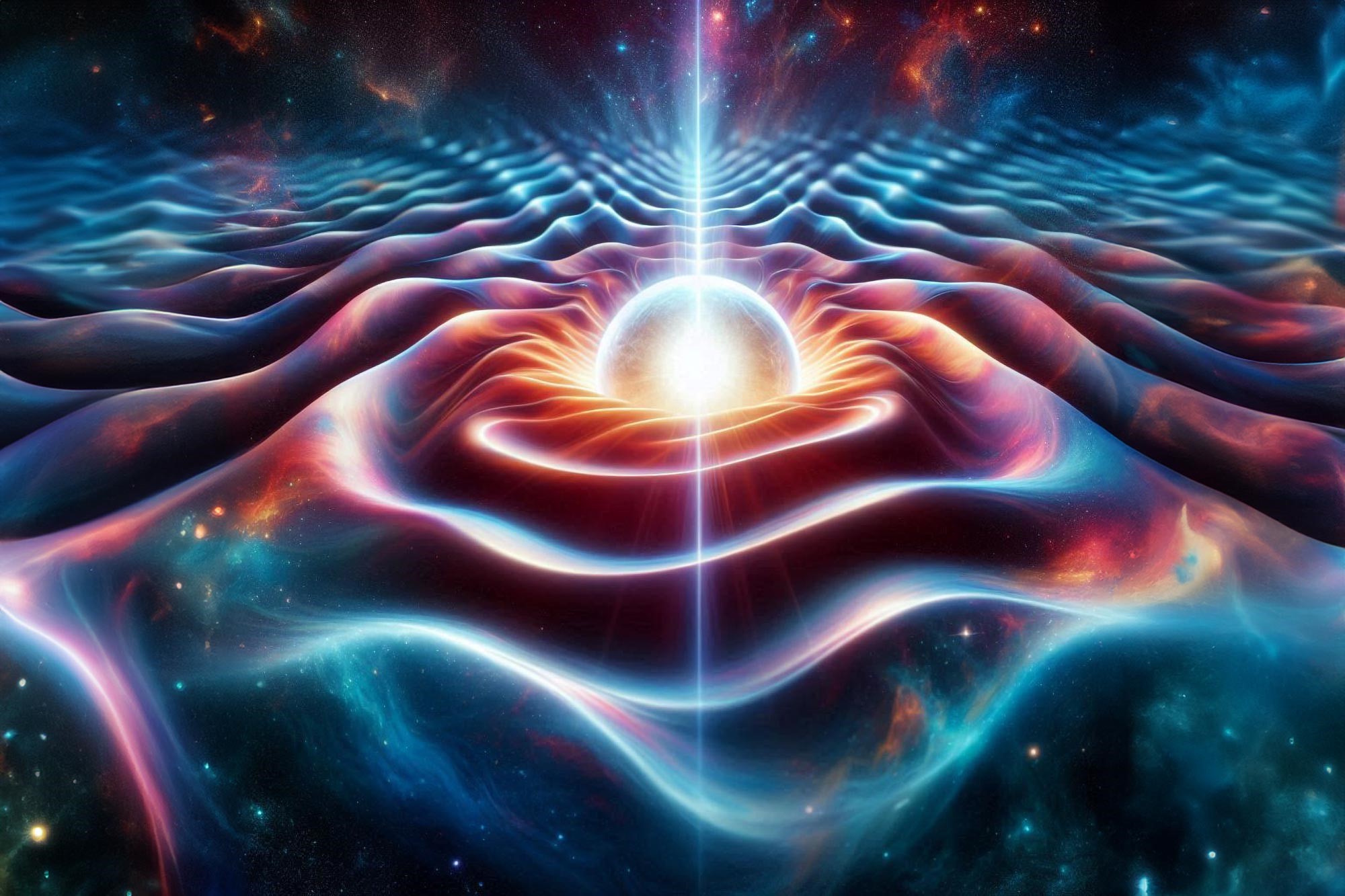
Figure 1. Machine Learning Enhances LIGO’s Signal Detection
LIGO: Unlocking the Universe’s Secrets
LIGO, the Laser Interferometer Gravitational-Wave Observatory, detects spacetime ripples from massive cosmic events. It was the first to observe gravitational waves from merging black holes, confirming Einstein’s Theory of Relativity. Comprising two 4-km-long interferometers in Hanford, Washington, and Livingston, Louisiana, LIGO uses high-powered lasers to capture these waves, offering insights into black holes, cosmology, and extreme states of matter. Thousands of data channels from environmental sensors help ensure detection accuracy by monitoring potential interference. Figure 1 shows Machine Learning Enhances LIGO’s Signal Detection.
Revolutionizing Noise Detection with Machine Learning
Jonathan Richardson, a UCR physicist leading the LIGO group, highlights how their machine learning tool autonomously identifies data patterns without human input. The system accurately recognizes environmental states known to LIGO operators, aiding in pinpointing noise sources and improving detector performance. Given LIGO’s extreme sensitivity to disturbances like ground motion, wind, and distant ocean waves, this approach helps mitigate glitches and enhance data quality.
Detecting Earthquakes, Microseisms, and Other Disturbances
LIGO continuously monitors environmental conditions using over 100,000 auxiliary channels with seismometers and accelerometers. The newly developed tool can identify various environmental states, including earthquakes, microseisms, and anthropogenic noise, across carefully curated sensing channels. Vagelis Papalexakis presented the team’s research on multivariate time series clustering for environmental state characterization at the IEEE’s 5th International Workshop on Big Data & AI Tools in Washington, D.C.
Uncovering Patterns with Machine Learning
The research team developed a machine learning model that autonomously identifies patterns in LIGO data, closely matching known environmental states recognized by human operators. Collaborating with the LIGO Scientific Collaboration, they secured a large dataset release, enabling further validation and algorithm development. Their findings reveal a strong link between external environmental noise and certain data glitches, offering potential solutions to mitigate these disturbances. The team spent a year organizing and analyzing LIGO’s extensive data channels, marking a significant effort in improving gravitational wave detection.
Advancing Machine Learning for Scientific Discovery
The UCR team led the release of a groundbreaking dataset on behalf of the LIGO Scientific Collaboration, which includes 3,200 members. This dataset is expected to significantly impact the machine learning and computer science communities. Their tool integrates signals from various environmental sensors at LIGO sites, distilling them into a single state that helps identify correlations between noise disturbances and environmental conditions, improving gravitational wave detection accuracy.
Striving for Practical Improvements in LIGO Detectors
By identifying patterns in noise, researchers can make physical modifications to LIGO detectors, such as replacing components, to improve performance [1]. Their machine learning tool aims to uncover new associations between environmental states and unknown noise issues. Additionally, the team successfully released a large-scale dataset, encouraging interdisciplinary research in data science and machine learning.
Reference:
- https://scitechdaily.com/breakthrough-ai-reveals-the-universes-hidden-signals/
Cite this article:
Janani R (2025), AI Breakthrough Uncovers Hidden Signals in the Universe, AnaTechMaz, pp. 586