Sentiment Analysis
Sentiment analysis, also known as opinion mining, is a natural language processing technique that involves analysing and classifying the polarity of subjective text such as reviews, comments, and social media posts, into positive, negative, or neutral categories. The goal of sentiment analysis is to extract the underlying sentiment or opinion expressed in the text.
Our increasingly digital world generates exponential amounts of data as audio, video, and text. While natural language processors are able to analyze large sources of data, they are unable to differentiate between positive, negative, or neutral speech. Moreover, when support agents interact with customers, they are able to adapt their conversation based on the customers’ emotional state which typical NLP model’s neglect. Therefore, startups are creating NLP models that understand the emotional or sentimental aspect of text data along with its context. Such NLP models improve customer loyalty and retention by delivering better services and customer experiences.[1]
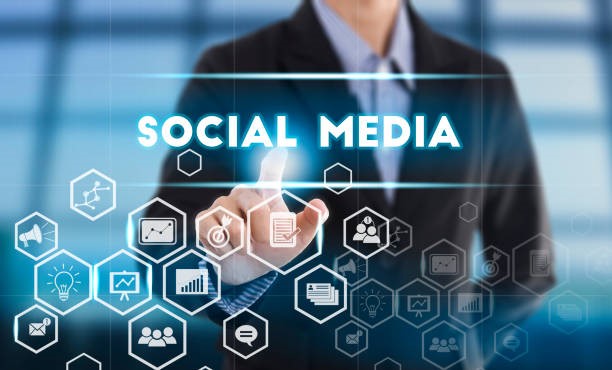
Figure .1 Sentiment analysis
Figure 1 shows Sentiment analysis can be approached using various techniques, such as rule-based methods, statistical models, and machine learning algorithms. Rule-based methods use pre-defined rules and dictionaries of sentiment words to classify the sentiment of a text. Statistical models and machine learning algorithms learn from annotated training data to classify the sentiment of text.
There are different levels of sentiment analysis: document-level, sentence-level, and aspect-based. Document-level sentiment analysis involves classifying the overall sentiment of a document, while sentence-level sentiment analysis involves classifying the sentiment of each individual sentence. Aspect-based sentiment analysis, on the other hand, involves identifying and classifying the sentiment of specific aspects or features of a product or service.
Sentiment analysis has various applications, including customer feedback analysis, brand monitoring, market research, and social media analysis. It can also be useful in industries such as e-commerce, healthcare, and finance.
One of the challenges of sentiment analysis is dealing with the nuances and complexities of natural language. For example, sarcasm, irony, and context can affect the polarity of a text. In addition, sentiment analysis models need to be trained on diverse and representative datasets to avoid bias and improve performance.
In summary, sentiment analysis is a natural language processing technique that involves analysing and classifying the polarity of subjective text into positive, negative, or neutral categories. Sentiment analysis can be approached using various techniques and has various applications in industries such as customer feedback analysis, brand monitoring, and market research. One of the challenges of sentiment analysis is dealing with the complexities of natural language and avoiding bias in the training data.
References:
- https://www.startus-insights.com/innovators-guide/natural-language-processing-trends/ - named-entity-recognition
Cite this article:
Janani R (2023), Sentiment analysis, AnaTechMaz, pp.236