Explainable AI
As machine learning algorithms are increasingly being used in decision-making processes, there is a growing demand for models that are transparent and can be easily explained. Explainable AI (XAI) is an area of research that focuses on developing algorithms that can provide clear and understandable explanations for their predictions.
In the last few years, Artificial Intelligence (AI) has achieved a notable momentum that, if harnessed appropriately, may deliver the best of expectations over many application sectors across the field. For this to occur shortly in Machine Learning, the entire community stands in front of the barrier of explain ability, an inherent problem of the latest techniques brought by sub-symbolism (e.g. ensembles or Deep Neural Networks) that were not present in the last hype of AI (namely, expert systems and rule based models).[1]
The literature on artificial intelligence (AI) or machine learning (ML) in mental health and psychiatry lacks consensus on what “explainability” means. In the more general XAI (eXplainable AI) literature, there has been some convergence on explainability meaning model-agnostic techniques that augment a complex model (with internal mechanics intractable for human understanding) with a simpler model argued to deliver results that humans can comprehend. Given the differing usage and intended meaning of the term “explainability” in AI and ML, we propose instead to approximate model/algorithm explainability by understandability defined as a function of transparency and interpretability.[2]
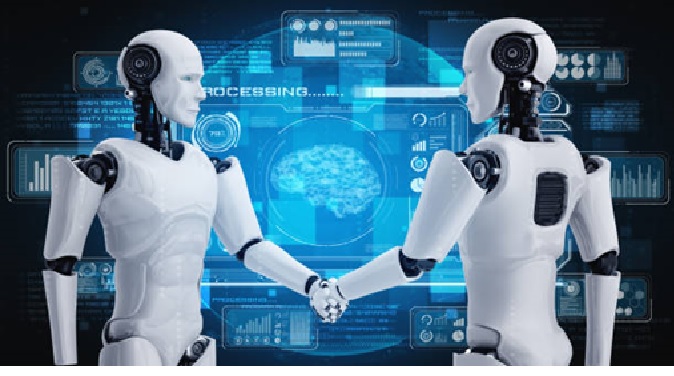
Figure .1 Explainable AI
Figure 1 shows Explainable AI (XAI) refers to the development of machine learning algorithms and models that can provide clear and understandable explanations for their predictions or decisions.
As machine learning becomes more prevalent in various industries and applications, there is a growing need to understand how and why machine learning models make certain predictions or decisions. However, many complex machine learning models, such as deep neural networks,can be difficult to interpret and explain, making it challenging to understand the underlying decision-making processes.
Explainable AI aims to address this issue by developing machine learning models that can provide interpretable and transparent explanations of their decisions. These explanations can help build trust in machine learning systems, enable users to understand the reasoning behind the model's predictions, and allow for better decision-making.
There are several approaches to building explainable AI models, including:
- Interpretable models: These are models that are designed to be easily interpretable and explainable. Examples include decision trees, rule-based models, and linear regression models.
- Model-agnostic methods: These are techniques that can be applied to any machine learning model to provide explanations for its predictions. Examples include feature importance scores, partial dependence plots, and LIME (Local Interpretable Model-Agnostic Explanations).
- Post-hoc explanations: These are explanations generated after the model has made its predictions. Examples include generating counterfactual explanations, which show how a small change in input data can lead to a different prediction, and generating natural language explanations.
Overall, explainable AI is an important area of research in machine learning, as it has the potential to improve the transparency and accountability of machine learning systems, and to help build trust in these systems among users and stakeholders
References:
- https://www.sciencedirect.com/science/article/abs/pii/S1566253519308103
- https://www.nature.com/articles/s41746-023-00751-9
Cite this article:
Janani R (2023),Explainable AI, AnaTechMaz,pp.209