Descriptive Analytics
Descriptive analytics is a type of data analysis that focuses on summarizing and describing past events or data to gain insights and understanding of what has happened. It deals with the analysis of historical data to identify patterns, trends, and correlations that can be used to understand the underlying causes and factors that influence a particular phenomenon.
Descriptive analytics is concerned with answering questions such as "what happened?" and "how did it happen?". It involves the use of statistical measures such as mean, median, mode, and standard deviation to summarize data and provide an overview of key characteristics and features.
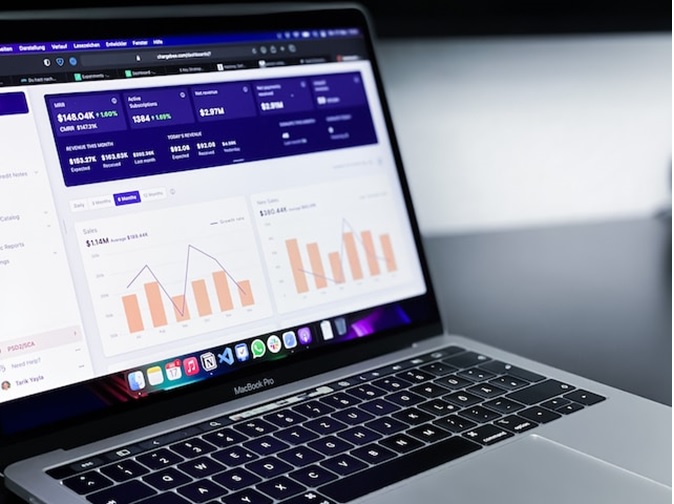
Figure 1. Descriptive Analytics
Figure 1 shows descriptive analytics. Descriptive analytics breaks down into five steps, including:
1. State the Business Metrics
For starters, the business must identify the metrics that it wants to generate based on the essential business goals of each group within the company or the company's overall goals. For instance, a company emphasizing growth may emphasize measuring quarterly revenue increases. At the same time, the company's accounts receivable department might monitor great days' sales and other metrics that show how much time it takes to collect money from their customers.
2. Identify the Data Required
Next, the company must find the data needed to generate the desired metrics. This task is a potential challenge since the relevant data may be scattered across many files and applications. However, companies that employ an Enterprise Resource Planning (ERP) system may have an easier time because they will already have most or all the needed data in their systems' databases. Furthermore, some metrics may also need data from external sources, like e-commerce websites, industry benchmarking databases, or social media platforms.
3. Extract and Prepare the Data
Extracting, combining, and preparing the relevant data for analysis is potentially time-consuming if the needed analysis data originates from multiple sources. However, this is a crucial step to ensure accuracy. Furthermore, this may involve data cleansing to eliminate inconsistencies and mistakes in the data, a reasonable effort considering the information coming from an eclectic group of sources and rendering data into a suitable format for analysis tools. Advanced data analytics types use a process known as data modeling, a framework residing within information systems to help prepare, arrange, and organize the company's information. Data modeling defines and formats complex data, turning it into a usable, actionable resource.
4. Analyze the Data
Companies have various tools at their disposal to apply descriptive analytics, ranging from business intelligence (BI) software to spreadsheets such as ones found in Excel. Descriptive analytics usually involves using fundamental mathematical operations to one or more of the variables. For instance, a sales manager might like to monitor the average sales revenue or the monthly revenue from either established or recently acquired customers.
5. Present the Data
Once business analysts have gone through the necessary steps, all that's left is presenting the data. First, however, the information must be presented so that everyone can understand it, from stakeholders to finance specialists. Stakeholders usually appreciate seeing the report in compelling visual forms, like bar charts, pie charts, or line graphs. Visible data is easier to grasp. Finance specialists on the other hand, may want the information presented through numbers and tables.
One example of descriptive analytics is the use of data visualization tools such as charts, graphs, and heat maps to represent patterns and trends in sales, customer behavior, or website traffic over a given period. Another example is the use of clustering algorithms to group customers into segments based on their purchasing behavior or demographic characteristics.
Descriptive analytics is a valuable tool for businesses and organizations to gain insights into their operations, identify opportunities for improvement, and make data-driven decisions. However, it is limited in its ability to provide predictive or prescriptive insights, which require more advanced analytics techniques.
References:
- https://www.simplilearn.com/what-is-descriptive-analytics-article#what_does_descriptive_analytics_tell_us
Cite this article:
Hana M (2023), Descriptive Analytics, AnaTechMaz, pp.46