Innovative Algorithm Enhances Tipping Point Prediction by Filtering Noisy Data
To anticipate critical thresholds, whether in climate patterns or mental health trends, mathematical analysis suggests monitoring fluctuations in data. University at Buffalo researchers have devised a groundbreaking algorithm outlined in Nature Communications. Harnessing stochastic differential equations, this algorithm pinpoints pivotal data points signaling proximity to tipping points, which could trigger irreversible changes. Lead author Naoki Masuda, along with co-authors Neil Maclaren and Kazuyuki Aihara, have pioneered an approach that not only identifies predictive data nodes but also considers their interconnections within networks.
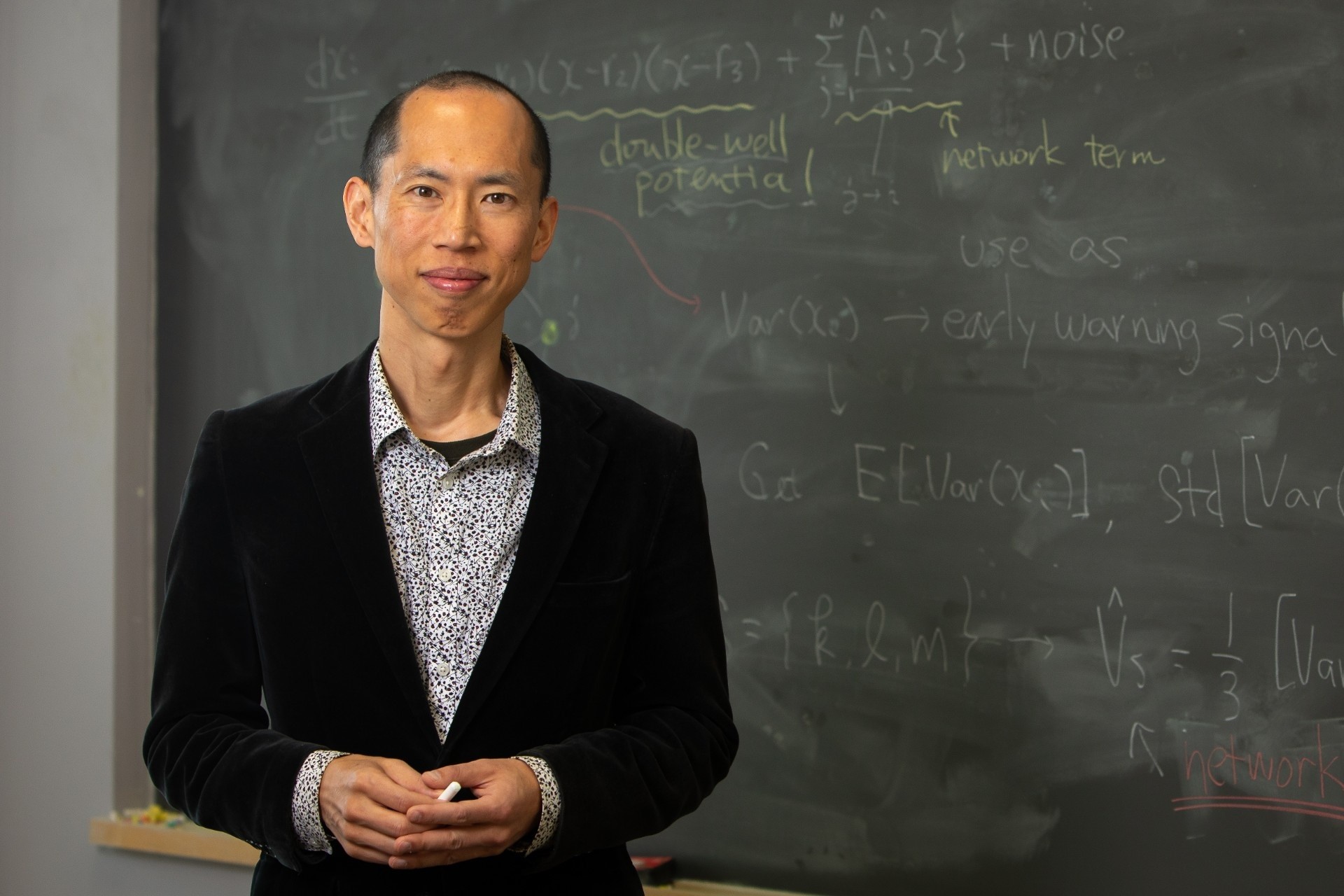
Figure 1. Naoki Masuda. (Credit: University at Buffalo)
Network-Based Approach to Early Warning Signals
Figure 1 shows Naoki Masuda. Traditionally, early warning systems have focused on individual data fluctuations. However, Masuda advocates for incorporating network science, especially relevant in fields like ecology and psychology where phenomena are interconnected [1]. For instance, in mental health research, symptoms often form a network, influencing each other and potentially indicating the onset of disorders. The algorithm devised by Masuda and his team goes beyond identifying high-fluctuation nodes, recognizing the importance of relationships between these nodes within a network.
Optimizing Early Warning Signals
Contrary to conventional wisdom, selecting nodes solely based on their individual fluctuations may not yield the most accurate signals. Masuda's team discovered that optimal early warning signals often stem from a combination of nodes with varying degrees of fluctuation [2]. This nuanced approach considers not only the individual node's signal quality but also its relationship with other selected nodes in the network.
Practical Application and Future Directions
What sets this algorithm apart is its applicability to real-world data without prior knowledge of network structures. Its reliance on just two states of the system makes it adaptable across diverse fields. Moving forward, Masuda and his team aim to collaborate with experts in ecology, climate science, and medicine to refine and test the algorithm using empirical data, fostering deeper insights into complex systems and potential tipping points.
Source: University at Buffalo
References:
- https://www.eurekalert.org/news-releases/1042722
- https://www.buffalo.edu/news/releases/2024/04/new-algorithm-cuts-through-noisy-data-to-better-predict-tipping-points.html
Cite this article:
Hana M (2024), Innovative Algorithm Enhances Tipping Point Prediction by Filtering Noisy Data, AnaTechMaz, pp. 255