Adversarially Robust: The Benefits of Peripheral Vision for Machines
Research from MIT suggests that a certain type of robust computer-vision model perceives visual representations similarly to the way humans do using peripheral vision. These models, known as adversarially robust models, are designed to overcome subtle bits of noise that have been added to image data.
The way these models learn to transform images is similar to some elements involved in human peripheral processing, the researchers found. But because machines do not have a visual periphery, little work on computer vision models has focused on peripheral processing, says senior author Arturo Deza, a postdoc in the Center for Brains, Minds, and Machines. [1]
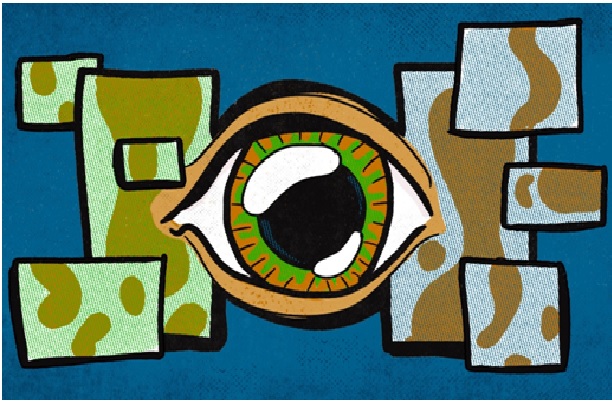
Figure 1. Adversarially Robust: The Benefits of Peripheral Vision for Machines
Figure 1 shows the results suggest that designing a machine-learning model to include some form of peripheral processing could enable the model to automatically learn visual representations that are robust to some subtle manipulations in image data. This work could also help shed some light on the goals of peripheral processing in humans, which are still not well-understood, Deza adds. [2]
Double vision
Humans and computer vision systems both have what is known as foveal vision, which is used for scrutinizing highly detailed objects. Humans also possess peripheral vision, which is used to organize a broad, spatial scene. Typical computer vision approaches attempt to model foveal vision — which is how a machine recognizes objects — and tend to ignore peripheral vision, Deza says.
But foveal computer vision systems are vulnerable to adversarial noise, which is added to image data by an attacker. In an adversarial attack, a malicious agent subtly modifies images so each pixel has been changed very slightly — a human wouldn’t notice the difference, but the noise is enough to fool a machine. [3]
Screen time
They started with a set of images and used three different computer vision models to synthesize representations of those images from noise: a “normal” machine-learning model, one that had been trained to be adversarially robust, and one that had been specifically designed to account for some aspects of human peripheral processing, called Texforms.
The team used these generated images in a series of experiments where participants were asked to distinguish between the original images and the representations synthesized by each model. Some experiments also had humans differentiate between different pairs of randomly synthesized images from the same models. [4]
References:
- https://techilive.in/the-benefits-of-peripheral-vision-for-machines/
- https://scitechdaily.com/adversarially-robust-the-benefits-of-peripheral-vision-for-machines/
- https://news.mit.edu/2022/machine-peripheral-vision-0302
- https://indiaeducationdiary.in/massachusetts-institute-of-technology-the-benefits-of-peripheral-vision-for-machines/
Cite this article:
Sri Vasagi K (2022), Adversarially Robust: The Benefits of Peripheral Vision for Machines, AnaTechMaz, pp. 99