BAFT AI Autosave System Reduces Training Losses by 98%
Researchers from Shanghai Jiao Tong University, Shanghai Qi Zhi Institution, and Huawei Technologies have developed BAFT, an advanced autosave system designed to reduce AI training downtime and enhance efficiency.
BAFT optimizes AI training by utilizing idle moments to improve fault tolerance and reduce computational overhead, establishing a new standard for reliable model development. The research is published in Frontiers of Computer Science.
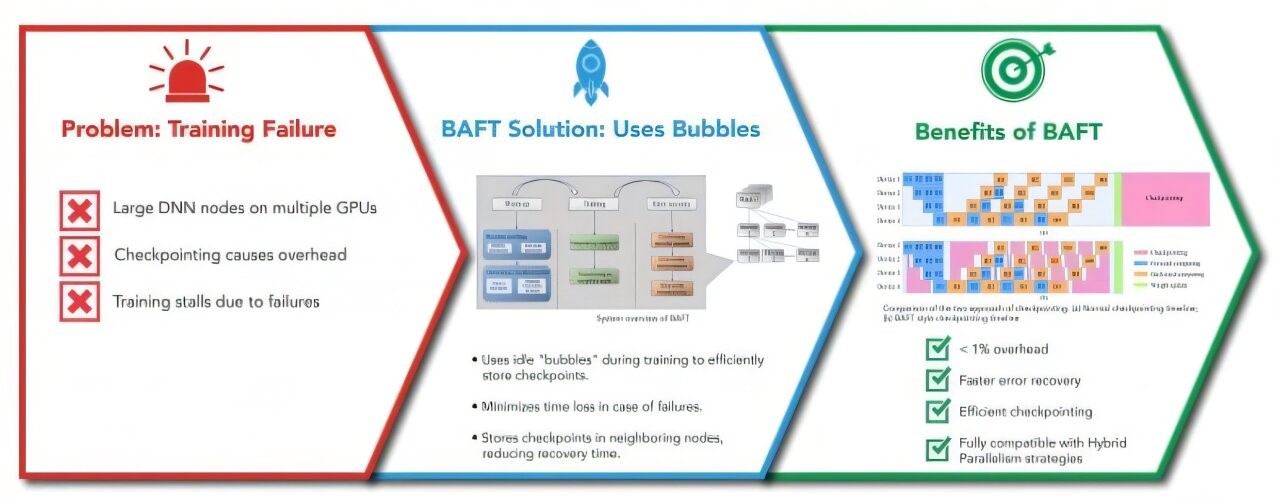
Figure 1. BAFT AI Cuts Training Losses By 98%
BAFT acts like an autosave feature for AI training, securing progress during idle moments or "bubbles." Unlike conventional checkpointing, it integrates seamlessly with less than 1% overhead, ensuring minimal disruption. Figure 1 shows BAFT AI cuts training losses by 98%.
BAFT enhances AI training by minimizing computational waste and improving fault tolerance. By leveraging idle moments, it optimizes resource use, ensuring continuous learning with minimal disruptions while maintaining accuracy and stability.
A reliable training process enables AI models to quickly recover from failures, minimizing lost progress and enhancing overall performance [1]. Unlike traditional systems, which risk setbacks from unexpected shutdowns or errors, this approach ensures continuity and efficiency.
BAFT minimizes training disruptions by enabling near-instant recovery, preventing significant data loss and ensuring a more reliable AI training process. Research shows that BAFT reduces training losses by 98%, making it one of the most efficient AI recovery systems to date.
Professor Minyi Guo of Shanghai Jiao Tong University emphasized the framework's impact, stating that it represents a major advancement in distributed AI training. He highlighted its practicality in maintaining the resilience of large-scale AI models, even amid unexpected system failures.
Key Advantages of BAFT:
- Minimal Downtime: Limits AI training losses to only 1–3 iterations (0.6–5.5 seconds) for smooth recovery.
- Optimized Performance: Utilizes snapshot transfers during idle periods, avoiding the 50% slowdown seen in traditional checkpointing methods.
- Scalable Across Industries: Strengthens AI model resilience in areas like autonomous driving, virtual assistants, and large-scale deep learning networks.
As AI becomes essential across industries, rapid recovery from system failures is critical. BAFT minimizes training disruptions, enabling organizations to scale AI operations efficiently while reducing costly downtime.
References:
- https://techxplore.com/news/2025-03-baft-ai-autosave-losses.html
Cite this article:
Janani R (2025), BAFT AI Autosave System Reduces Training Losses by 98%, AnaTechMaz, pp. 344