Machine Learning
Machine learning is the concept that a computer program can learn and adapt to new data without human intervention. Machine learning is a field of artificial intelligence (AI) that keeps a computer’s built-in algorithms current regardless of changes in the worldwide economy.
In the real world, we are surrounded by humans who can learn everything from their experiences with their learning capability, and we have computers or machines which work on our instructions. But can a machine also learn from experiences or past data [1] like a human does? So here comes the role of Machine Learning.
Machine Learning is said as a subset of artificial intelligence that is mainly concerned with the development of algorithms which allow a computer to learn from the data and past experiences on their own. The term machine learning was first introduced by Arthur Samuel in 1959. We can define it in a summarized way as figure1.
Machine learning enables a machine to automatically learn from data, improve performance from experiences, and predict things without being explicitly programmed.
With the help of sample historical data, which is known as training data, machine learning algorithms build a mathematical model that helps in making predictions or decisions without being explicitly programmed. Machine learning brings computer science and statistics together for creating predictive models. Machine learning constructs or uses the algorithms that learn from historical data. The more we will provide the information, the higher will be the performance.
- 1. Machine learning is an area of artificial intelligence (AI) with a concept that a computer program can learn and adapt to new data without human intervention.
- 2. A complex algorithm or source code is built into a computer that allows for the machine to identify data and build predictions around the data that it identifies.
- 3. Machine learning is useful in parsing the immense amount of information that is consistently and readily available in the world to assist in decision making.
- 4. Machine learning can be applied in a variety of areas, such as in investing, advertising, lending, organizing news, fraud detection, and more.
Uses of Machine Learning
Machine learning is used in different sectors for various reasons. Trading systems can be calibrated to identify new investment opportunities. Marketing and e-commerce platforms can be tuned to provide accurate and personalized recommendations to their users based on the users’ internet search history or previous transactions[2]. Lending institutions can incorporate machine learning to predict bad loans and build a credit risk model. Information hubs can use machine learning to cover huge amounts of news stories from all corners of the world. Banks can create fraud detection tools from machine learning techniques. The incorporation of machine learning in the digital-savvy era is endless as businesses and governments become more aware of the opportunities that big data presents.
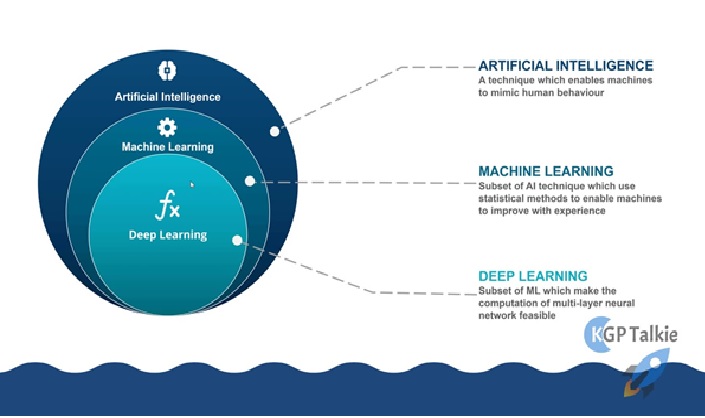
Figure 1: Machine learning have three types of learning sector.
Understanding Machine Learning
Various sectors of the economy are dealing with huge amounts of data available in different formats from disparate sources. The enormous amount of data, known as big data, is becoming easily available and accessible due to the progressive use of technology, specifically advanced computing capabilities and cloud storage. Companies and governments realize the huge insights that can be gained from tapping into big data but lack the resources and time required to comb through its wealth of information [3]. As such, artificial intelligence measures are being employed by different industries to gather, process, communicate, and share useful information from data sets. One method of AI that is increasingly utilized for big data processing is machine learning.
The various data applications of machine learning are formed through a complex algorithm or source code built into the machine or computer. This programming code creates a model that identifies the data and builds predictions around the data it identifies. The model uses parameters built in the algorithm to form patterns for its decision-making process. When new or additional data becomes available, the algorithm automatically adjusts the parameters to check for a pattern change, if any. However, the model shouldn’t change.
Application of Machine Learning
How machine learning works can be better explained by an illustration in the financial world. Traditionally, investment players in the securities market like financial researchers, analysts, asset managers, and individual investors scour through a lot of information from different companies around the world to make profitable investment decisions. However, some pertinent information may not be widely publicized by the media and may be privy to only a select few who have the advantage of being employees of the company or residents of the country where the information stems from. In addition, there’s only so much information humans can collect and process within a given time frame. This is where machine learning comes in.
An asset management firm may employ machine learning in its investment analysis and research area. Say the asset manager only invests in mining stocks. The model built into the system scans the web and collects all types of news events from businesses, industries, cities, and countries, and this information gathered makes up the data set. The asset managers and researchers of the firm [4] would not have been able to get the information in the data set using their human powers and intellects. The parameters built alongside the model extracts only data about mining companies, regulatory policies on the exploration sector, and political events in select countries from the data set.
Example of Machine Learning
Say mining company XYZ just discovered a diamond mine in a small town in South Africa. A machine learning tool in the hands of an asset manager that focuses on mining companies would highlight this as relevant data. The model in the machine learning tool would then use an analytics tool called predictive analytics to make predictions on whether the mining industry will be profitable for a time period, or which mining stocks are likely to increase in value at a certain time, based on the recent information discovered, without any input from the asset manager. This information is relayed to the asset manager to analyze and make a decision for their portfolio. The asset manager may then make a decision to invest millions of dollars into XYZ stock.
In the wake of an unfavorable event, such as South African miners going on strike, the computer algorithm adjusts its parameters automatically to create a new pattern. This way, the computational model built into the machine stays current even with changes in world events and without needing a human to tweak its code to reflect the changes. Because the asset manager received this new data on time, they are able to limit their losses by exiting the stock figure2.
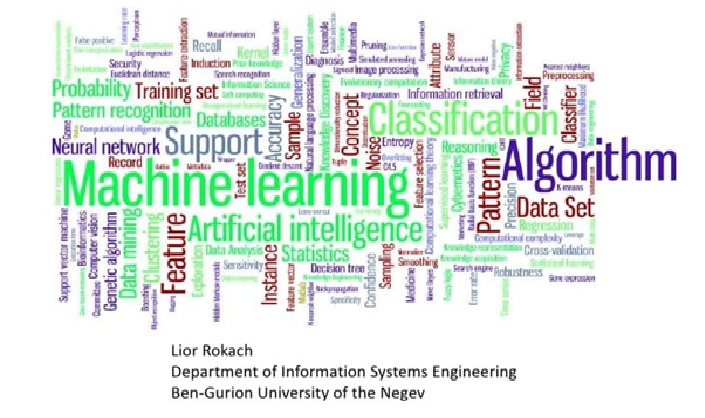
Figure 2: The full content in machine learning in this diagram.
References:
- Whats mean by maching learning its definition? Reterived at april from 2020 www.investopedia.com/terms/m/machine-learning.asp
- https://www.ibm.com/cloud/learn/machine-learning
- https://en.wikipedia.org/wiki/Machine_learning
- https://www.investopedia.com/terms/m/machine-learning.asp
Cite this article:
D. Vinotha (2021), Machine learning, AnaTechmaz, pp. 2