Quantum Machine Learning
Quantum machine learning (QML) is an emerging field that combines quantum computing with machine learning algorithms to give solutions for problems that are very difficult to solve in a conventional way. Quantum machine learning uses quantum computers for training machine learning models. Quantum computers can process data in a special way using quantum bits (qubits) instead of conventional bits used by classical digital computers. Quantum machine learning has emerged due to the [1] current issues with big data processing. Machine learning models can be trained in a faster way using quantum computing. In addition, using Quantum algorithms, large spaces can be searched for approximating the functions.
Quantum machine learning can be defined as the technology wherein quantum algorithms are applied to machine learning problems. The goal is to find the best possible solution of a given quantum algorithm for a particular problem and apply it in real-world applications like image recognition, language translation, or any other task which can be solved using machine learning. Quantum algorithms figure 1 shows could help transform artificial intelligence (AI)/machine learning (ML) use cases by accelerating big data analytics at incredible speeds.
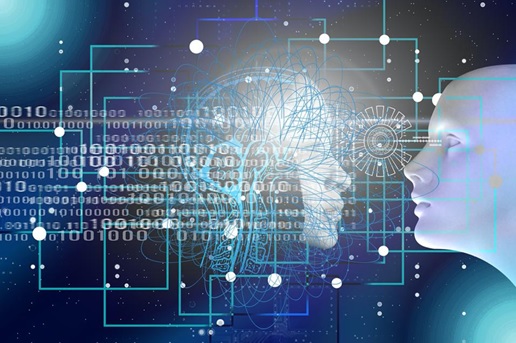
Figure1: Quantum Machine Learning
Boost of machine learning
Machine learning is a branch of the computer sciences designed to quickly parse through big data while using predictive analysis to create responses to human queries. One benefit of quantum science is that this new [2] method of computing allows for better data classifications. Machine learning algorithms become “smarter” or at least more accurate in their decision-making based on the data you’re feeding it. Quantum computing will allow machine learning algorithms to identify data patterns that are currently invisible to traditional computers.
Just imagine the world’s fastest computer combined with self-learning algorithms — the implications for research, healthcare, aerospace, economics, and other areas with currently untenable problems are staggering. SingularityHub suggests quantum computers are very good at simulating nature. There are implications for climate change and drug research, as well as materials science and information transfer. But the truth is, we don’t know exactly what these machines will eventually do because we’re not sure we are even asking the right questions.
Limitation of machine learning
Hardware limitations
The frequent challenge that troubles researchers is isolation. Quantum decoherence can be caused by heat and light, when subjected to such condition’s qubits can lose their quantum properties like entanglement [3] that further leads to a loss in data stored in these qubits. Secondly, rotations in quantum computers’ logic gates are prone to error and these are also crucial to change the state of the qubit. Any wrong rotation can cause an error in the output.
Software limitations
The developer of algorithms for Quantum computers has to be concerned about their physics. While a classical algorithm can be developed along the lines of the Turing machine, to develop an algorithm for Quantum computers, the developer has to base it along the lines of raw physics with no simple formulas that would link it to logic.
References:
- https://vitalflux.com/quantum-machine-learning-concepts-and-examples/
- https://www.windzr.com/blog/benefits-applying-quantum-computing-machine-learning
- https://analyticsindiamag.com/exploring-the-limitations-of-quantum-machine-learning/
Cite this article:
S. Nandhinidwaraka (2021) Quantum Machine Learning, AnaTechmaz, pp.8