Harvard-Developed Clinical AI Performs on Par with Human Radiologists
Scientists from Harvard Medical School and Stanford University have created a diagnostic tool using artificial intelligence that can detect diseases on chest x-rays based on natural language descriptions provided in attached clinical reports.
Since most existing AI models require arduous human annotation of huge amounts of data before labeled data is fed into the model to train it, this step is considered a big step forward in clinical design. of AI. [1]
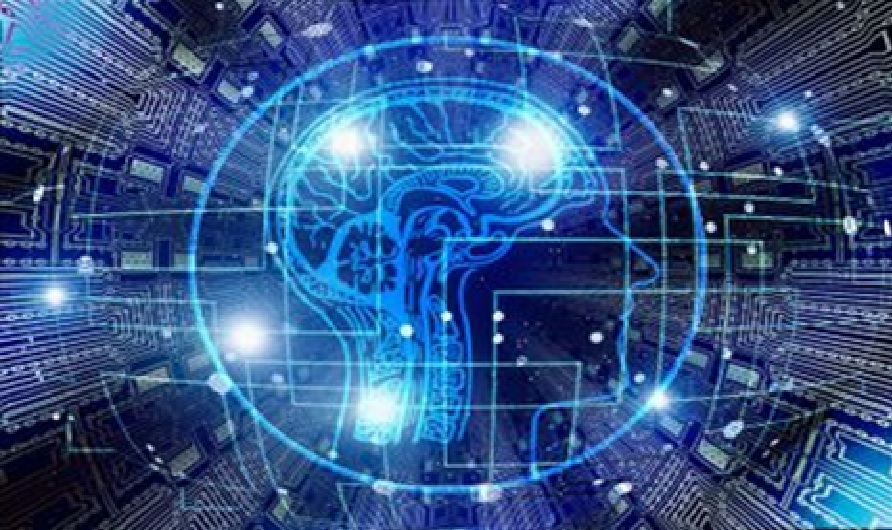
Figure 1. Harvard-Developed Clinical AI Performs on Par with Human Radiologists
Figure 1 shows the mannequin, named CheXzero, carried out on par with human radiologists in its potential to determine pathologies on chest X-rays, in line with a paper describing their work that was printed in Nature Biomedical Engineering. The group has additionally made the mannequin’s code brazenly accessible to different researchers.
To appropriately detect pathologies throughout their “coaching,” the vast majority of AI algorithms want labeled datasets. Since this process requires in depth, typically pricey, and time-consuming annotation by human clinicians, it’s significantly tough for duties involving the interpretation of medical photographs.
For example, to label a chest X-ray dataset, skilled radiologists must take a look at a whole bunch of hundreds of X-ray photographs one after the other and explicitly annotate every one with the circumstances detected. Whereas more moderen AI fashions have tried to handle this labeling bottleneck by studying from unlabeled knowledge in a “pre-training” stage, they ultimately require fine-tuning on labeled knowledge to attain excessive efficiency. [2]
By contrast, the new model is self-supervised, in the sense that it learns more independently, without the need for hand-labeled data before or after training. The model relies solely on chest X-rays and the English-language notes found in accompanying X-ray reports.
The model was “trained” on a publicly available dataset containing more than 377,000 chest X-rays and more than 227,000 corresponding clinical notes. Its performance was then tested on two separate datasets of chest X-rays and corresponding notes collected from two different institutions, one of which was in a different country. This diversity of datasets was meant to ensure that the model performed equally well when exposed to clinical notes that may use different terminology to describe the same finding. [3]
References:
- https://vademogirl.com/2022/11/05/cbmix2h0dhbzoi8vc2npdgvjagrhawx5lmnvbs9oyxj2yxjklwrldmvsb3blzc1jbgluawnhbc1has1wzxjmb3jtcy1vbi1wyxitd2l0ac1odw1hbi1yywrpb2xvz2lzdhmv0gfjahr0chm6ly9zy2l0zwnozgfpbhkuy29tl2hhcnzhcmqtzgv2zwxvcgvklwnsaw5p/
- https://www.gnflearning.com/harvard-developed-medical-ai-performs-on-par-with-human-radiologists/
- https://scitechdaily.com/harvard-developed-clinical-ai-performs-on-par-with-human-radiologists/
Cite this article:
Thanusri swetha J, Harvard-Developed Clinical AI Performs on Par with Human Radiologists, Anatechmaz, pp.188