A deep learning technique to generate DSN reinforcement attacks
Deep learning techniques have recently shown promise for detecting cybersecurity attacks and determining their nature. At the same time, many cybercriminals have devised new attacks to disrupt the operation of various deep learning tools, including those for image classification and natural language processing.
Perhaps the most common of these attacks are hostile attacks, which are designed to fool deep learning algorithms using data that has changed, causing them to misclassify it. This can lead to failures in many applications, biometric systems and other technologies that operate through deep learning algorithms.[1]
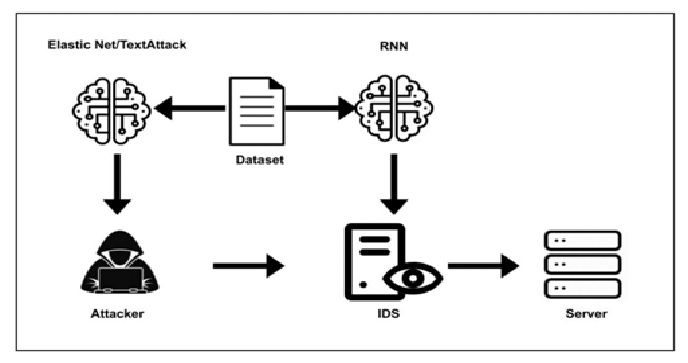
Figure 1. A deep learning technique to generate DSN reinforcement attacks
Figure 1 shows several previous studies have shown the effectiveness of various adversary attacks to induce deep neural networks (DNNs) to make unreliable and false predictions. These attacks include the Carlini & Wagner attack, the Deepfool attack, the fast gradient drawing method (FGSM), and the Elastic-Net attack (ENA). [1]
The scale of Internet-connected systems has increased considerably, and these systems are being exposed to cyber attacks more than ever. The complexity and dynamics of cyber attacks require protecting mechanisms to be responsive, adaptive, and large-scale. Machine learning, or more specifically deep reinforcement learning (DRL), methods have been proposed widely to address these issues. By incorporating deep learning into traditional RL, DRL is highly capable of solving complex, dynamic, and especially high-dimensional cyber defense problems. [2]
Researchers at the Citadel have recently developed a DNN that can detect a type of cyberattack known as distributed denial of service (DDoS) DSN amplification, and then used two different algorithms to generate adversarial examples that could trick their DNN. Their findings, published in a paper pre-published on arXiv, further confirm the unreliability of deep learning methods for DSN attack detection and their vulnerability to adversarial attacks.
In the future, the work by this team of researchers at the Citadel could inspire the development of more effective tools for detecting DDoS DSN amplification attacks, which can detect adversarial data and correctly classify it. In their next studies, the researchers plan to test the effectiveness of adversarial attacks on a particular type of algorithms for detecting DNS amplification attacks, those targeting the so-called constrained application protocol (CoAP) used by many IoT devices. [3]
References:
- https://badpi.com/a-deep-learning-technique-to-generate-dsn-reinforcement-attacks/
- https://www.researchgate.net/publication/333773807_Deep_Reinforcement_Learning_for_Cyber_Security
- https://newsazi.com/a-deep-learning-technique-to-generate-dsn-amplification-attacks/
Cite this article:
Thanusri swetha J (2022), A deep learning technique to generate DSN reinforcement attacks, AnaTechMaz, pp.255