AI Techniques in Image and Object Analysis
In archaeology, the analysis of images and objects plays a crucial role in understanding past civilizations. AI techniques have revolutionized this aspect of archaeological research, enabling automated classification, detection, and segmentation of archaeological artifacts and features. This summary highlights the key AI techniques used in image and object analysis in archaeology.
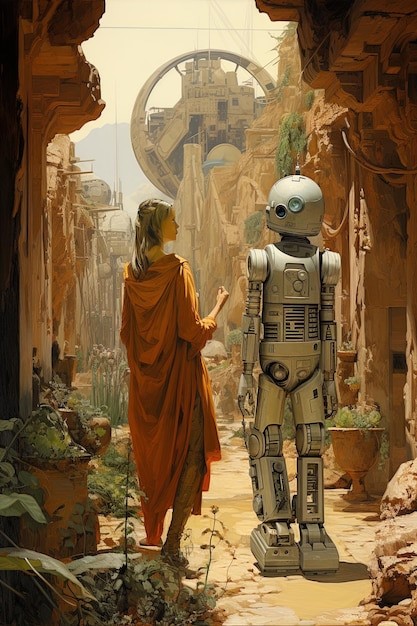
Figure 1. AI Techniques in Image and Object Analysis
Image Classification:
Convolutional Neural Networks (CNNs):
Figure 1 Shows AI Techniques in Image and Object Analysis. CNNs are powerful deep learning models for image analysis, capable of automatically learning features from images.
In archaeology, CNNs are used to classify artifacts, architectural features, and site layouts based on their visual characteristics.
Example: Classifying pottery types or architectural styles from excavation photographs.
Transfer Learning:
Transfer learning involves fine-tuning pre-trained CNN models on archaeological datasets, reducing the need for large labeled datasets.
This approach enables efficient training of accurate image classification models even with limited archaeological data.
Object Detection:
Region-Based CNNs (R-CNNs):
R-CNNs and their variants (e.g., Fast R-CNN, Faster R-CNN) are used for object detection in archaeological images.
These models generate region proposals and classify objects within those regions, enabling accurate detection of artifacts and features.
Example: Detecting and classifying archaeological artifacts in excavation photographs.
You Only Look Once (YOLO) and Single Shot Multibox Detector (SSD):
YOLO and SSD are real-time object detection algorithms suitable for archaeological applications where speed is crucial.
These models provide fast and accurate detection of objects in archaeological images, facilitating rapid analysis of large datasets.
Example: Identifying and cataloging artifacts in real-time during excavation.
Segmentation:
Semantic Segmentation:
Semantic segmentation techniques divide archaeological images into meaningful regions, enabling the identification and classification of different elements within the scene.
This approach is used to delineate artifacts, structures, and landscape features in archaeological photographs.
Example: Segmenting excavation images to separate artifacts from background clutter.
Instance Segmentation:
Instance segmentation goes a step further by not only segmenting objects but also distinguishing between individual instances of the same object class.
This technique is valuable for counting and analyzing individual artifacts and features within archaeological images.
Example: Counting and measuring pottery fragments in excavation photographs.
Practical Applications:
Artifact Classification and Cataloging:
AI facilitates the automated classification and cataloging of artifacts based on their visual characteristics, streamlining the archaeological analysis process.
This allows archaeologists to efficiently manage large artifact collections and identify patterns in material culture.
Site Mapping and Feature Detection:
AI techniques enable the automated mapping and detection of archaeological features such as structures, roads, and settlement boundaries from satellite imagery and aerial surveys.
This aids in the identification and documentation of archaeological sites over large geographic areas.
AI techniques in image and object analysis have transformed archaeological research by providing powerful tools for automated classification, detection, and segmentation of artifacts and features. By leveraging deep learning models such as CNNs and R-CNNs, archaeologists can analyze large datasets of excavation photographs, satellite imagery, and 3D scans with unprecedented speed and accuracy.[1] These AI-driven approaches enhance our understanding of past civilizations and contribute to more efficient archaeological exploration and conservation efforts.
References:
- https://www.linkedin.com/pulse/applications-ai-archaeology-unveiling-hidden-secrets-david-cain-eb2qc
Cite this article:
Janani R (2024), AI and Archaeology: Discoveries and Insights from Data Analysis, Anatechmaz, pp.3