'Self-driving' microscopes discover shortcuts to new materials
Researchers at the Department of Energy’s Oak Ridge National Laboratory are teaching microscopes to drive discoveries with an intuitive algorithm, developed at the lab’s Center for Nanophase Materials Sciences, that could guide breakthroughs in new materials for energy technologies, sensing and computing.
“There are so many potential materials, some of which we cannot study at all with conventional tools, that need more efficient and systematic approaches to design and synthesize,” said Maxim Ziatdinov of ORNL’s Computational Sciences and Engineering Division and the CNMS. “We can use smart automation to access unexplored materials as well as create a shareable, reproducible path to discoveries that have not previously been possible.” [1]
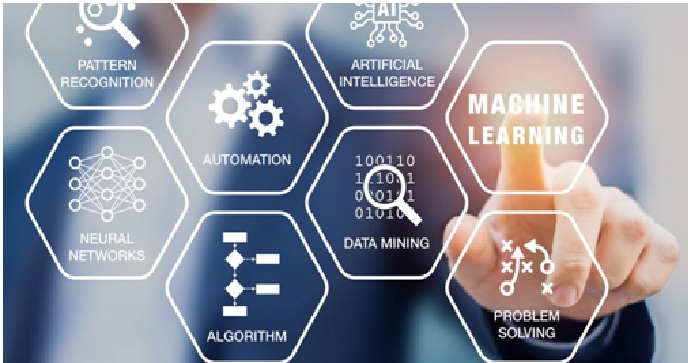
Figure 1. 'Self-driving' microscopes discover shortcuts to new materials
Figure 1 shows the approach, published in Nature Machine Intelligence, combines physics and machine learning to automate microscopy experiments designed to study materials' functional properties at the nanoscale. [2]
For a smarter automation approach, the ORNL workflow incorporates human-based physical reasoning into machine learning methods and uses very small amounts of data – images acquired from less than 1% of the sample – as a starting point. The algorithm selects points of interest based on what it learns within the experiment and on knowledge outside the experiment.
As proof of the concept, a workflow was demonstrated using scanning probe microscopy and applied to well-studied ferroelectric materials. Ferroelectrics are functional materials with an unorientable surface charge that can be used for calculation, activation and sensing applications. Researchers are interested in understanding the link between the amount of energy or information that these materials can store and the local domain structure that governs this property. The automated experiment discovered the specific topological defects for which these parameters are optimized. [3]
“The takeaway is that the workflow was applied to material systems familiar to the research community and made a fundamental finding, something not previously known, very quickly—in this case, within a few hours,” Ziatdinov said.
Results were faster—by orders of magnitude—than conventional workflows and represent a new direction in smart automation. [4]
References:
- https://techiai.com/self-driving-microscopes-discover-shortcuts-to-new-materials/
- https://www.sciencedaily.com/releases/2022/05/220509150750.htm
- https://www.tamilbloggers.xyz/self-driving-microscopes-discover-shortcuts-to-new-materials/
- https://www.labmanager.com/news/self-driving-microscopes-discover-shortcuts-to-new-materials-28080
Cite this article:
Thanusri swetha J (2022), 'Self-driving' microscopes discover shortcuts to new materials, Anatechmaz, pp.92