Controlling Stickiness of Bandages Using Waves and Sounds
Tracking underwater targets using autonomous underwater vehicles (AUVs) poses a significant challenge due to the complex environment and uncertain motion patterns. This issue hampers model-based control approaches. To overcome this, the problem is treated as a Markov decision process (MDP) with unknown state transitions. A solution is presented using an actor-critic framework and experience replay technique, resulting in a model-free reinforcement learning algorithm. To enhance its effectiveness, an adaptive experience replay scheme is introduced.
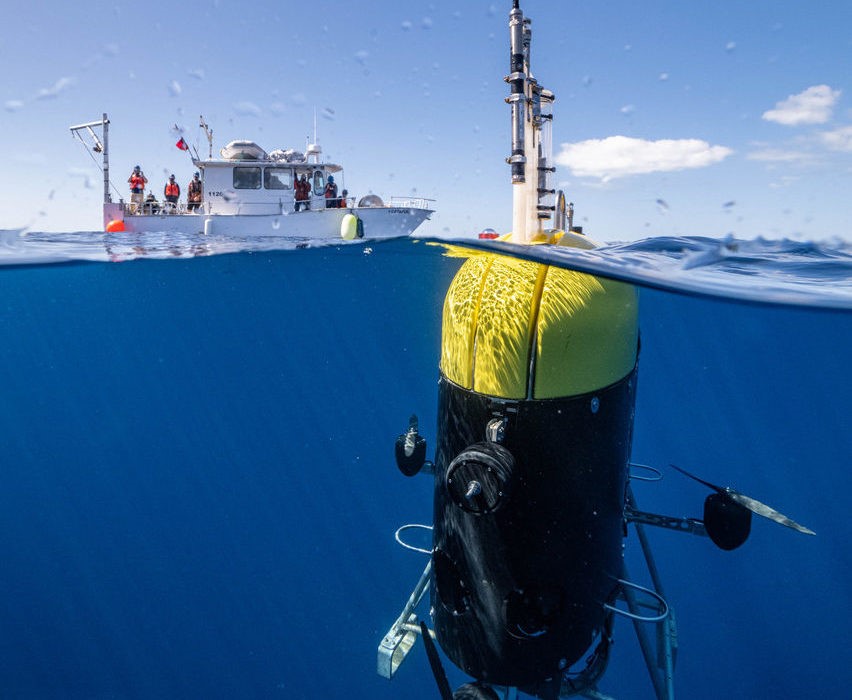
Figure 1. Reinforcement Learning for Dynamic Underwater Robotic Target Tracking
Figure 1 shows the algorithm leverages an experience replay buffer to store and shuffle samples, allowing for training neural networks using time series data.[1] This approach addresses the complexities of underwater target tracking for AUVs, offering a dynamic solution that is adaptable to the uncertainties of the underwater environment.
To harness the potential of autonomous underwater robots and expand our oceanic observation capabilities, novel techniques are essential. Employing fleets of autonomous robots holds promise for studying intricate marine ecosystems and creatures through innovative imaging setups or by monitoring tagged animals to understand their behaviours. These insights can then guide the formulation of conservation policies for the community.[2] A significant knowledge gap lies in comprehending animal connectivity; particularly how active animal movement influences the distribution of deep-sea populations.
Efficiently tracking underwater targets poses a substantial challenge for studying biological processes in their natural habitat. Consequently, the development of methods that can adeptly respond to dynamic environmental changes during monitoring missions becomes crucial. The strategic placement of sensors and the optimal planning of paths to locate underwater targets are intricate tasks requiring specialized analytical techniques, particularly in complex scenarios.
The primary objective of this study was to explore the potential of reinforcement learning as a tool for optimizing range-only underwater target tracking, leveraging its successful application in terrestrial scenarios. The study aimed to assess the effectiveness of reinforcement learning by implementing it as a path planning system for an autonomous surface vehicle tasked with tracking an underwater mobile target. The research encompasses a comprehensive explanation of an open-source model, assessment metrics in simulated environments, and the evaluation of algorithms based on extensive field experiments conducted at sea, spanning over 15 hours.
The culmination of these endeavours highlights the potency of deep reinforcement learning as an approach that enhances the capabilities of autonomous oceanic robots. This study underscores the viability of deploying such algorithms for monitoring marine biological systems, thus paving the way for more effective exploration and understanding of the ocean's complexities in the future.
References:
- https://www.mdpi.com/2077-1312/10/10/1406
- https://www.science.org/doi/10.1126/scirobotics.ade7811
Cite this article:
Janani R (2023), Reinforcement Learning for Dynamic Underwater Robotic Target Tracking,Anatechmaz, pp.480