Scientists Uncover a Hidden Superpower in Microscopes Using AI
The illustration shows how the new microscopy method works: light travels through the microscope, and due to chromatic aberration, red, green, and blue wavelengths focus at different distances. As light passes through the specimen, its phase changes—captured as separate color images by a standard camera. An AI model then calculates these phase shifts for enhanced image analysis.
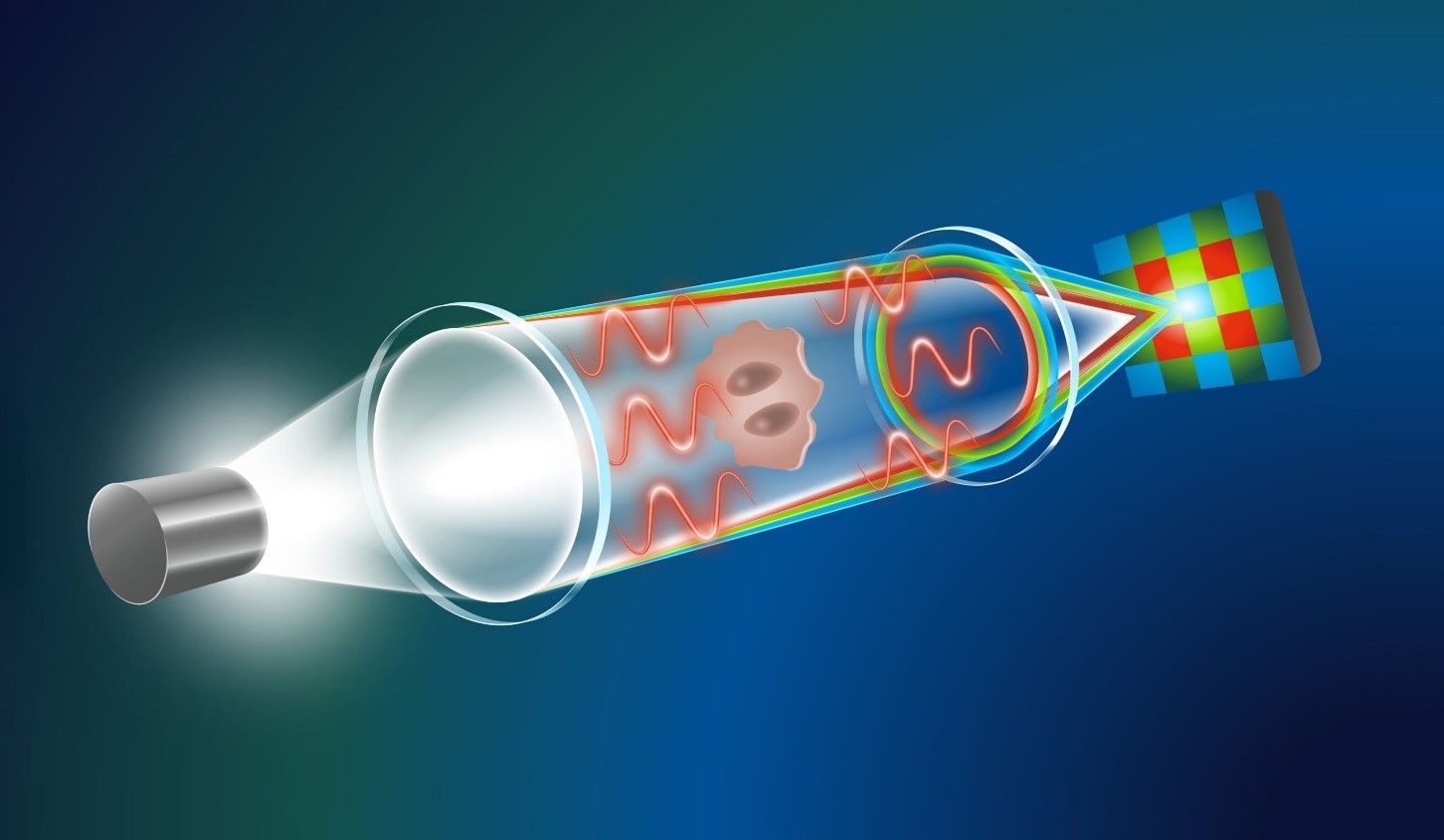
Figure 1. Microscopes AI.
Traditional microscopy often requires expensive, time-consuming staining with dyes. In contrast, this innovative computational quantitative phase imaging (QPI) technique uses the natural focal differences of light wavelengths to generate through-focus image stacks from a single exposure. By applying a specially trained diffusion model, it produces high-quality images of biological specimens, such as red blood cells, offering a cost-effective, efficient alternative for medical diagnostics. Microscopes AI shown in figure 1.
Unveiling Biological Details Without the Need for Labels
Labeling biological samples with dyes or staining agents provides critical insights, but it comes with notable limitations in clinical diagnostics, such as high costs, lengthy processing times, and the need for specialized equipment. To overcome these challenges, researchers are increasingly exploring label-free microscopy techniques like quantitative phase imaging (QPI).
Unlike conventional imaging methods that rely on light absorption or scattering, QPI captures how a sample alters the phase of light passing through it [1]. This phase shift reveals valuable information about the sample’s thickness, refractive index, and structural composition. While traditional QPI setups often require advanced and expensive instruments, computational QPI offers a more affordable and accessible solution, opening new possibilities for fast and efficient biological imaging.
The Power of Computational QPI
A prominent computational QPI technique is based on solving the Transport-of-Intensity Equation (TIE), a mathematical approach that reconstructs high-quality images by analyzing the phase changes introduced as light passes through a sample. This method can be easily integrated into standard optical microscopes, making it a practical option for enhancing biological imaging.
However, a significant limitation of the TIE method is its reliance on capturing multiple images at varying focal distances to reduce imaging artifacts. This process can be time-consuming and technically demanding, posing challenges for real-time clinical applications. As a result, the need for faster, more efficient computational QPI methods has driven researchers to explore AI-powered solutions.
Utilizing Chromatic Aberration for Imaging
“Our approach relies on the similar principles as TIE but only needs one image because of a clever combination of physics and generative AI,” says Prof. Artur Yakimovich, Leader of a CASUS Young Investigator Group and corresponding author of the work presented at the AAAI Conference. The information about the phase shift induced by biological specimen does not come from additional exposures taken with other focus distances.
A through-focus stack can also be generated from one single exposure thanks to a phenomenon called chromatic aberration. Most lens systems of the microscope cannot bring all wavelengths of (polychromatic) white light to a single converging point perfectly – a handicap that only highly specialized lenses can correct. This means e.g. red, green and blue (RGB) light have slightly different focus distances.
“By recording the phase shifts of those three wavelengths separately using a conventional RGB detector, one can build a through-focus stack that facilitates computational QPI turning the handicap into an asset,” Yakimovich explains.
AI Solves Major Challenges
“Utilizing chromatic aberration for QPI presents a significant challenge: the focal distance between red and blue light is extremely small,” explains Gabriel della Maggiora, a PhD student at CASUS and one of the two lead authors of the study. This minimal distance makes it difficult to obtain meaningful results using the conventional TIE approach.
“We then considered leveraging artificial intelligence to overcome this limitation — a decision that proved to be crucial,” della Maggiora adds. “By training a generative AI model on an open-access dataset of 1.2 million images, the model successfully extracted phase information from the limited data captured during a single exposure, significantly enhancing the imaging quality.”
Method Successfully Tested on Real-World Clinical Specimens
Inspired by last spring’s Conditional Variational Diffusion Model (CVDM)—which delivers high-quality results with less computational effort—the team developed a novel diffusion model for quantitative data. This breakthrough enabled them to implement computational QPI using chromatic aberrations and validate the approach on real clinical specimens [2]. Using a standard brightfield microscope with a commercial color camera, their AI-driven method revealed the donut-like shape of red blood cells in a human urine sample—details that traditional TIE-based methods missed—while eliminating cloud artifacts. The Yakimovich group “Machine Learning for Infection and Disease” is advancing computational microscopy for immediate clinical applications by integrating physics-based elements into generative AI to reduce hallucinations.
Reference:
- https://scitechdaily.com/scientists-just-discovered-a-hidden-superpower-in-microscopes-thanks-to-ai/
- https://lifeboat.com/blog/2025/03/scientists-just-discovered-a-hidden-superpower-in-microscopes-thanks-to-ai
Cite this article:
Keerthana S (2025),Scientists Uncover a Hidden Superpower in Microscopes Using AI,, AnaTechmaz, pp.1103.