A Causal Framework for Investigating Gene Cause-And-Effect Relationships
By studying changes in gene expression, researchers can gain insights into how cells function at a molecular level, potentially leading to a better understanding of disease development.
However, with approximately 20,000 genes in humans, each interacting in complex ways, identifying the right genes to target is an immensely challenging task. Genes also work in modules that regulate each other, further complicating the process.
Researchers at MIT have now laid the theoretical groundwork for methods that could effectively group genes into related clusters, allowing scientists to uncover the underlying cause-and-effect relationships between genes.
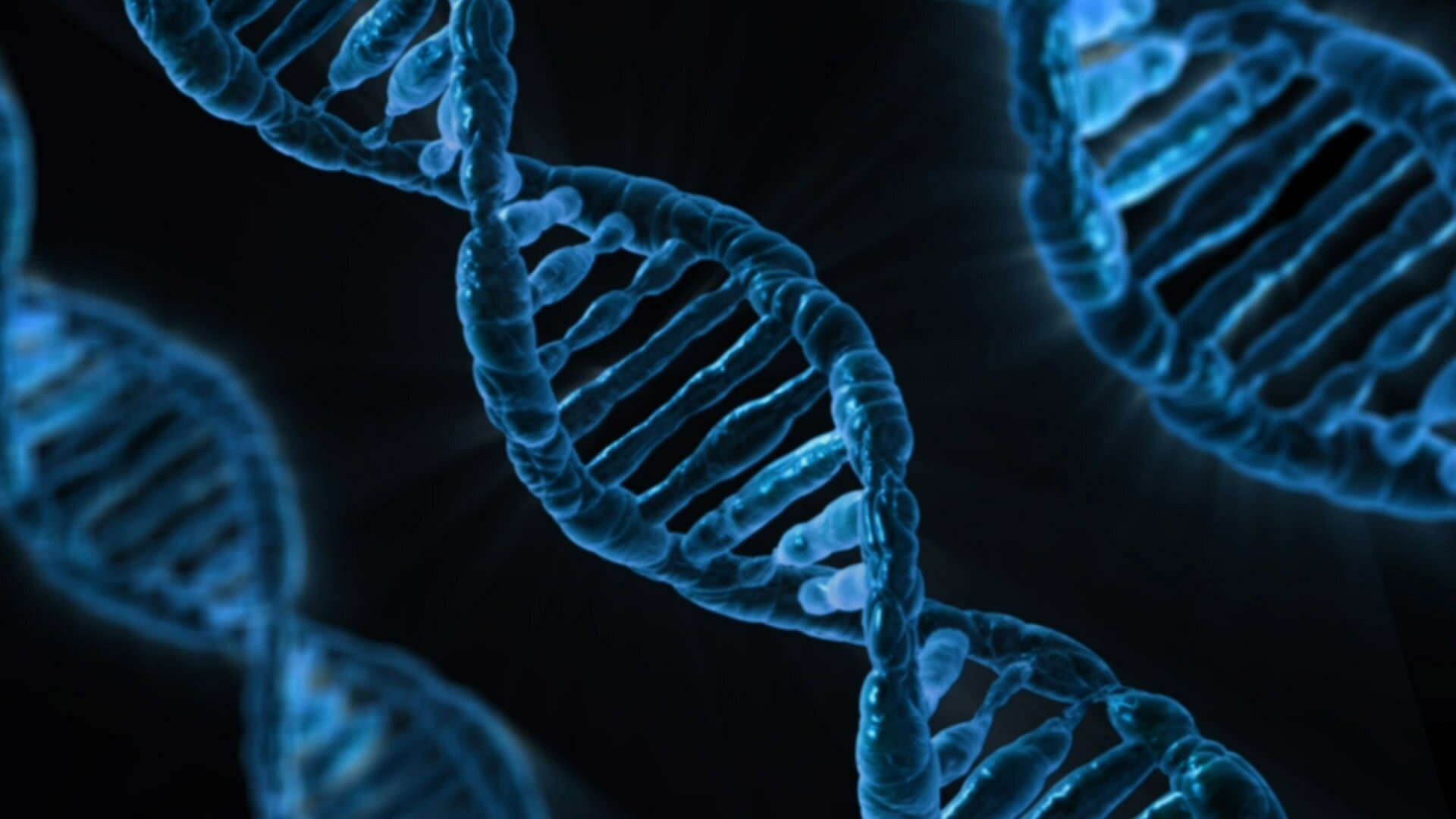
Figure 1. Causal Theory for Gene Relationships
Crucially, this new method uses only observational data, meaning researchers don’t need to conduct costly or sometimes impractical interventional experiments to gather the data needed to infer causal links. In the future, this approach could help scientists more accurately and efficiently identify gene targets, leading to more precise treatments for patients.Figure 1 shows Causal Theory for Gene Relationships
MIT researchers, including graduate student Jiaqi Zhang, have developed a new method for identifying the best way to group genes for studying complex gene relationships in cells. By aggregating genes into related groups, this method helps researchers more effectively understand the cause-and-effect relationships between genes, even without the need for costly intervention-based experiments. The approach promises to enhance the identification of gene targets and could potentially lead to more precise treatments for diseases by improving the understanding of cell states at a molecular level.
Gaining Insights from Observational Data
The researchers aimed to address the challenge of learning gene programs, which describe how groups of genes work together to regulate biological processes like cell development or differentiation.
Given the complexity of studying all 20,000 genes and their interactions, scientists use causal disentanglement to group related genes in a way that facilitates the exploration of cause-and-effect relationships. In previous studies, the team demonstrated the effectiveness of this approach when interventional data (from experiments that manipulate variables) were available.
However, conducting interventional experiments is costly, and there are situations where such experiments may be unethical or not feasible with current technology.
With only observational data, researchers cannot directly compare the effects of gene interventions. “Most research in causal disentanglement assumes access to interventions, so it was unclear how much could be learned from observational data alone,” Zhang explains.
To overcome this, the MIT team developed a more general approach using machine learning algorithms to identify and group observed variables, like genes, based on observational data. This method enables the identification of causal modules and the reconstruction of the underlying cause-and-effect mechanisms. "While this work was initially driven by the need to understand cellular programs, we first had to create new causal theories to determine what could and couldn't be learned from observational data. With these insights, future research can help identify gene modules and their regulatory relationships," says Uhler.
Inferring Insights from Observational Data
The researchers aimed to address the challenge of uncovering gene programs, which describe how genes collaborate to regulate one another in biological processes like cell development or differentiation.
Because studying the interactions of all 20,000 genes is not feasible, they employ a method called causal disentanglement. This technique helps group related genes together in a way that allows for the efficient exploration of cause-and-effect relationships.
In previous research, the team demonstrated how this method could be applied effectively using interventional data, which are collected by altering variables within the network. However, conducting interventional experiments can be costly, and in some cases, they may be impractical or unethical due to limitations in technology.
Without intervention, researchers cannot directly compare gene behaviors before and after changes, making it difficult to understand how gene groups interact.
“Most research in causal disentanglement assumes that interventions are possible, so it was unclear how much could be understood from just observational data,” says Zhang.
To address this, the MIT team developed a more generalized approach using a machine-learning algorithm that aggregates groups of observed variables, such as genes, purely from observational data.
This technique allows them to identify causal modules and reconstruct an accurate model of underlying cause-and-effect mechanisms. “Our research was driven by the challenge of understanding cellular programs, but before tackling genetic data, we had to establish new causal theory to determine what could and couldn’t be learned from observational data. With this foundation, we plan to apply this knowledge to identify gene modules and their regulatory connections in future research,” says Uhler.
A Step-By-Step Representation
Using statistical methods, the researchers calculate a mathematical function known as the variance for the Jacobian of each variable's score. Causal variables that don't influence subsequent variables should have zero variance.
They then rebuild the representation layer by layer, beginning with the removal of variables in the bottom layer that have zero variance. Working backward, they continue removing variables with zero variance to identify which variables or groups of genes are linked.
"Identifying the variances that are zero becomes a complex combinatorial challenge, so developing an efficient algorithm to solve it was a significant hurdle," says Zhang.
Ultimately, their approach generates an abstracted representation of the observed data, organized into layers of interconnected variables that accurately capture the underlying cause-and-effect structure.
Each variable represents an aggregated group of genes working together, and the relationship between two variables signifies how one group of genes regulates another. The method successfully encapsulates all the information used to determine each layer of variables.
After demonstrating the theoretical validity of their technique, the researchers ran simulations to show that the algorithm can effectively disentangle meaningful causal representations using only observational data.
Looking ahead, the researchers aim to apply this technique in real-world genetic studies. They also seek to explore how their approach might offer additional insights when partial interventional data are available or help scientists design more effective genetic interventions. In the future, this method could aid researchers in more efficiently identifying gene programs and discovering drugs targeting these genes to treat specific diseases.
Source: MIT News
Cite this article:
Janani R (2024), A Causal Framework for Investigating Gene Cause-And-Effect Relationships, AnaTechmaz, pp.1043